comprehensive guide to Imitation Learning: Teaching AI by Demonstration 2024
Introduction
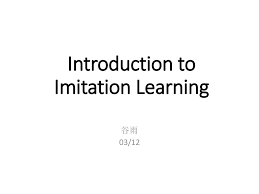
Imitation Learning (IL) is a machine learning approach where an AI agent learns by observing expert demonstrations rather than through trial-and-error reinforcement learning. This method is widely used in robotics, self-driving cars, gaming AI, and healthcare, where training an agent from scratch is inefficient or impractical.
This blog explores how imitation learning works, its key algorithms, and real-world applications.
1. What is Imitation Learning?
Imitation Learning enables an AI system to learn decision-making strategies from expert demonstrations instead of interacting with an environment through reinforcement learning.
πΉ Key Elements of Imitation Learning: β Expert Demonstrations β The AI system watches an expert perform a task.
β State-Action Pairs β The AI maps each state to an action.
β Policy Learning β The AI generalizes from expert behavior to new situations.
π Example: AI for Self-Driving Cars
β AI observes human drivers and learns how to steer, accelerate, and brake in different environments.
β Over time, AI models mimic human driving behavior with high accuracy.
β Imitation Learning accelerates AI training by leveraging human expertise.
2. Behavioral Cloning: The Simplest Form of Imitation Learning
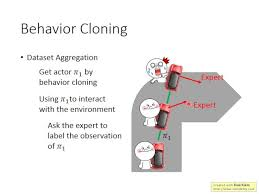
Behavioral Cloning (BC) is a supervised learning approach where an AI learns to directly imitate an expertβs actions.
πΉ How Behavioral Cloning Works: 1οΈβ£ Collect demonstrations from human experts.
2οΈβ£ Train a neural network to predict actions given a state.
3οΈβ£ Deploy the AI agent to perform tasks based on learned behavior.
π Formula for Policy Learning in Behavioral Cloning:Ο(aβ£s)=P(aβ£s,ΞΈ)Ο(a|s) = P(a | s, ΞΈ) Ο(aβ£s)=P(aβ£s,ΞΈ)
where Ο is the policy and ΞΈ are learned model parameters.
π Example: AI in Video Games
β AI learns turning angles and acceleration patterns from human players.
β Used in autonomous racing simulators and robotics competitions.
β Behavioral Cloning is effective but suffers from compounding errors when encountering new situations.
3. Challenges in Behavioral Cloning
While BC is simple and effective, it has three major limitations:
π§ 1. Compounding Errors
- If the AI makes a slight mistake, it can deviate from expert demonstrations, leading to irrecoverable errors.
π§ 2. Poor Generalization
- AI struggles with unseen scenarios (e.g., a self-driving car trained in sunny weather fails in the rain).
π§ 3. No Exploration
- The AI never learns beyond the expertβs actions, limiting its ability to adapt to new challenges.
π Key Challenge: How do we teach AI to correct its mistakes and generalize better?
β
Solution: Use advanced imitation learning methods like DAGGER and Inverse Reinforcement Learning (IRL).
4. DAGGER: A More Robust Imitation Learning Approach
DAGGER (Dataset Aggregation) improves on behavioral cloning by allowing the AI to request expert corrections when it makes mistakes.
β How DAGGER Works:
β Train the AI using behavioral cloning.
β Let the AI perform tasks autonomously.
β If the AI makes mistakes, the expert provides corrections.
β The AI updates its policy using both its own actions and expert feedback.
π Example: AI-Powered Drone Flying
β A drone learns to fly autonomously but makes mistakes.
β A human pilot provides corrections, improving the AIβs robustness.
β DAGGER allows interactive learning, making AI more adaptable.
5. Inverse Reinforcement Learning (IRL): Learning the Reward Function
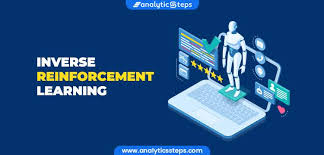
Inverse Reinforcement Learning (IRL) is a powerful approach where an AI infers the expertβs reward function from observed demonstrations.
β Why Use IRL?
- In standard RL, we define a reward function manually.
- In IRL, the AI learns what the expert values and optimizes accordingly.
π οΈ How IRL Works
β The AI observes expert demonstrations.
β It infers a hidden reward function that explains the observed behavior.
β It optimizes its policy using reinforcement learning (RL).
π Example: AI for Traffic Optimization
β AI watches human traffic controllers manage traffic lights.
β It learns hidden patterns and optimizes traffic flow autonomously.
β IRL enables AI to generalize expert behaviors to new situations.
6. Generative Adversarial Imitation Learning (GAIL): Combining GANs and IRL
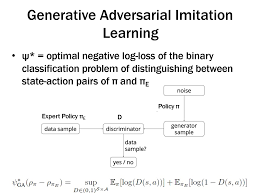
Recent research has combined IRL with Generative Adversarial Networks (GANs) to improve learning efficiency.
β How GAIL Works
1οΈβ£ Generator (AI policy) tries to imitate expert behavior.
2οΈβ£ Discriminator (Evaluator) tries to distinguish between expert actions and AI actions.
3οΈβ£ Feedback Loop: The AI improves until it is indistinguishable from expert behavior.
π Example: AI for Motion Capture Animation
β AI learns realistic human movement by imitating dancers and athletes.
β Used in film animation and virtual reality.
β GAIL helps AI achieve expert-level performance in highly dynamic tasks.
7. Applications of Imitation Learning
Imitation learning is transforming AI-driven automation in various industries.
Industry | Use Case |
---|---|
Autonomous Vehicles | AI mimics human driving for safer self-driving cars |
Healthcare AI | AI-assisted robotic surgery |
Robotics | AI-powered robotic arms learn manual tasks |
Gaming AI | AI NPCs learn player behavior for realistic interactions |
Finance | AI learns stock trading strategies from expert investors |
π Example: AI in Healthcare
β AI-assisted surgery robots learn from human surgeons.
β Used in AI-powered robotic surgical assistants.
β Imitation Learning makes AI more intuitive and human-like.
8. When to Use Imitation Learning?
Scenario | Best Approach |
---|---|
AI needs to mimic human expertise | Behavioral Cloning |
AI must recover from mistakes | DAGGER (Dataset Aggregation) |
AI must generalize expert behavior | Inverse Reinforcement Learning (IRL) |
AI needs to generate human-like actions | GAIL (Generative Adversarial Imitation Learning) |
π Key Takeaway:
Use Imitation Learning when mistakes are costly or exploration is dangerous.
9. Final Thoughts: The Future of Imitation Learning
Imitation Learning is revolutionizing AI, allowing systems to learn efficiently from human expertise rather than relying on random exploration.
π Key Takeaways
β Behavioral Cloning is effective but suffers from compounding errors.
β DAGGER improves AI learning by actively incorporating expert corrections.
β Inverse Reinforcement Learning (IRL) lets AI infer expert intentions.
β GAIL refines AIβs ability to mimic human behavior using adversarial training.
β Imitation Learning is widely used in robotics, self-driving cars, and gaming AI.
π‘ Whatβs Next?
Future research in imitation learning + reinforcement learning will create AI systems capable of autonomous decision-making in dynamic environments.
π Do you think imitation learning will lead to fully autonomous AI? Letβs discuss in the comments! π