comprehensive guide to Parallelizing Deep Neural Networks: Optimizing Performance for Large-Scale Training 2024
Deep learning has revolutionized many fields by enabling powerful models that can learn from vast amounts of data. However, as models grow in size and complexity, the time required to train them can become prohibitive. This is where parallelizing deep neural networks (DNNs) comes into play. By distributing the computational workload across multiple processing units (CPUs, GPUs, or even multiple nodes), we can significantly speed up training and optimize performance. In this blog, we will explore the key techniques and challenges associated with parallelizing deep learning models, and how they can be leveraged for optimal performance.
The Challenge of Training Deep Neural Networks
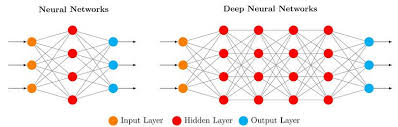
Training a deep neural network (DNN) typically involves two stages:
- Forward pass: The network processes input data, passing it through layers to produce an output.
- Backward pass (Backpropagation): The network adjusts its parameters based on the error (loss) derived from the output, using gradients calculated through backpropagation.
While the forward pass can often be evaluated quickly, the backward pass is far more computationally intensive. The problem becomes even more pronounced when scaling models to millions of parameters or when dealing with large datasets, making training time stretch from hours to days or weeks. This is where parallelization comes in.
Understanding Parallelization in DNNs
The goal of parallelizing DNNs is to reduce training time by distributing the computational load across multiple resources. There are two main ways to parallelize DNN training:
- Data Parallelism
- Model Parallelism
1. Data Parallelism
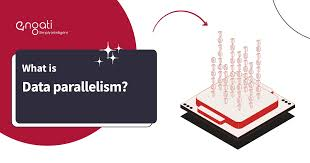
Data parallelism involves distributing the training dataset across multiple processing units (e.g., GPUs or machines). Each processing unit computes the forward and backward pass for a subset of the data. After the gradient computation, these gradients are aggregated and averaged across all units, and the model parameters are updated.
- Advantages:
- Efficient for large datasets.
- Ideal for scenarios where the model fits within the memory of a single processing unit but the dataset is too large to be processed by one machine.
- Challenges:
- Synchronizing and averaging gradients across all units introduces communication overhead.
- As the number of units increases, the communication cost may outweigh the benefits of parallelism.
2. Model Parallelism
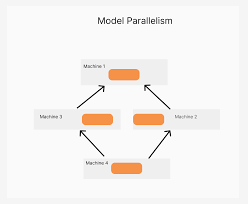
Model parallelism involves splitting the model itself across different processing units. Each unit handles a part of the model, and the data flows through these parts during both the forward and backward passes. This is particularly useful for large models that cannot fit entirely in the memory of a single processing unit.
- Advantages:
- Suitable for very large models that exceed the memory capacity of a single machine.
- Allows distributing different parts of the model (e.g., layers) across different devices.
- Challenges:
- Requires careful management of data flow between the different parts of the model.
- Can lead to bottlenecks as the layers must communicate frequently during training.
Hybrid Parallelism
In many cases, hybrid parallelism is used, combining both data and model parallelism. This hybrid approach maximizes the use of available hardware resources by splitting both the data and the model across multiple units. While this approach is more complex, it allows deep neural networks to scale efficiently across large datasets and complex models.
Training DNNs on GPUs
GPUs are particularly well-suited for deep learning tasks due to their high arithmetic intensity and ability to process many parallel operations. DNN operations, particularly matrix multiplications (which are common in neural networks), benefit greatly from GPUs, which are optimized for such tasks.
Libraries like cuDNN (CUDA Deep Neural Network) provide optimized GPU kernels for common operations in deep learning, such as convolution, activation functions, and pooling. These libraries enable deep learning models to achieve up to 10× speedup in training compared to CPU-based systems.
Challenges of Parallelizing DNNs
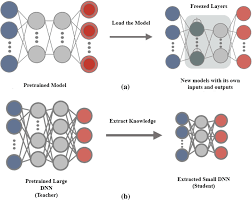
While parallelizing deep neural networks can significantly reduce training time, it also introduces several challenges that need to be carefully managed:
- Synchronization Overhead: As the number of processing units increases, the time spent synchronizing gradients and model parameters increases. Efficient communication strategies are crucial to minimize this overhead.
- Memory Management: Large models require careful memory management to ensure that the memory capacity of each processing unit is utilized efficiently. For model parallelism, this becomes even more important as different parts of the model are distributed across multiple units.
- Workload Imbalance: Different layers or units in a DNN may require varying amounts of computation. Load balancing becomes crucial to ensure that all processing units are utilized effectively without some units sitting idle while others are overloaded.
- Fault Tolerance: In distributed training systems, a failure in one processing unit can disrupt the entire training process. Implementing fault-tolerant systems is essential, particularly when scaling across many nodes.
Emerging Architectures for Parallel Deep Learning
To address these challenges, various specialized architectures and systems have emerged:
- NVIDIA Pascal and Volta GPUs: These GPUs are designed to accelerate deep learning tasks by supporting 16-bit floating-point operations and providing high memory bandwidth.
- Google’s TensorFlow Processing Unit (TPU): A hardware accelerator designed specifically for tensor computations. TPUs are optimized for deep learning tasks, providing a substantial boost in performance compared to general-purpose GPUs.
- Apple Neural Engine: Found in Apple’s A11 and A12 chips, these processors are designed for accelerating machine learning tasks on iPhones and iPads.
- Microsoft’s BrainWave: A project based on FPGAs (Field-Programmable Gate Arrays), which are optimized for real-time deep learning processing in data centers.
Future Directions and Optimizations
Future improvements in parallel DNN training may come from further advancements in hardware (such as ASICs, FPGAs, and TPUs), better interconnects for distributed computing, and algorithmic innovations. One potential direction is the development of specialized deep learning architectures that reduce the reliance on traditional hardware and offer more efficient ways to train large models in parallel.
Additionally, optimization techniques like pruning (removing unnecessary parameters) and quantization (reducing the precision of weights) are becoming increasingly popular as they help reduce the memory footprint of large models, enabling them to be trained more efficiently across distributed systems.
Conclusion
Parallelizing deep neural networks is essential for tackling the computational challenges posed by large-scale training tasks. By employing data parallelism, model parallelism, and hybrid approaches, training times can be dramatically reduced, making it feasible to train more complex and larger models. Leveraging specialized hardware like GPUs, TPUs, and FPGAs can further accelerate training and allow for real-time deep learning applications.
As deep learning continues to evolve, understanding and optimizing parallelism will be crucial to pushing the boundaries of AI and enabling more powerful and efficient machine learning models. The ongoing development of distributed training frameworks and hardware accelerators will be key to making deep learning accessible for increasingly demanding applications.