Understanding Big O, Big Theta, and Big Omega for Optimizing Algorithm Efficiency 2024
In the world of computer science and programming, understanding how to evaluate and compare the performance of algorithms is essential. Big O, Big Theta, and Big Omega are notations used to describe an algorithm’s time and space complexity. These notations help developers quantify the efficiency of their code and optimize it for real-world applications. While these terms might sound intimidating at first, they represent different aspects of algorithm performance, and understanding them is key to writing efficient software.
In this blog, we will dive into the differences between Big O, Big Theta, and Big Omega, using relatable examples and insights to clarify how each one applies to algorithmic analysis.
What Is Big O Notation?
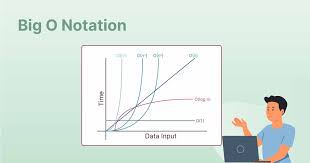
Big O notation (O) is the most commonly used of the three notations. It describes the worst-case performance of an algorithm, providing an upper bound on how slow the algorithm can be as the input size increases. Essentially, Big O gives you an idea of the worst scenario for an algorithm’s growth.
Imagine you’re in charge of delivering pizzas to guests at several parties (🎉). As the number of guests (n) increases, so does the time it takes to deliver the pizzas. If each guest is at a different party and requires a separate pizza, you are dealing with an O(n²) situation, where time increases exponentially as the number of guests increases.
Key Points:
- Big O describes the upper bound of the algorithm’s runtime.
- It’s particularly useful for analyzing worst-case scenarios, ensuring your app performs optimally even in the most demanding situations.
What Is Big Theta Notation?
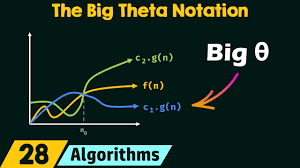
Big Theta notation (Θ) represents the average-case performance of an algorithm, providing a more balanced view than Big O. It describes an algorithm’s time complexity when both the upper and lower bounds of performance are the same. In other words, it’s used to represent the “typical” case, where the algorithm performs as expected on average.
Using our pizza delivery example, assume there are multiple guests at each party, and the time to deliver the pizzas scales more slowly with the number of guests (n). In this case, Big Theta notation might represent a linear relationship like Θ(n×p), where p is constant and increases proportionally with n, meaning the time to deliver pizzas increases linearly with the number of guests.
Key Points:
- Big Theta is the “sweet spot” between the best and worst-case performance.
- It’s useful for understanding the expected behavior of an algorithm in real-world scenarios.
What Is Big Omega Notation?
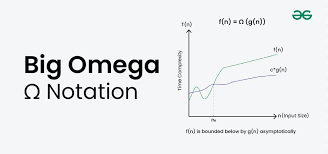
Big Omega notation (Ω) expresses the best-case performance of an algorithm, providing a lower bound on how fast the algorithm can run. It describes the ideal scenario where the algorithm runs as efficiently as possible, without any hindrances.
In our pizza party scenario, imagine all guests (n) are at a single party, so only one pizza needs to be delivered. In this best-case situation, the time complexity would be Ω(n), scaling linearly with the number of guests, since only one delivery is needed.
Key Points:
- Big Omega represents the best case performance, showing the minimum amount of time an algorithm requires to run.
- It helps determine how fast the algorithm can perform when conditions are ideal.
When to Use Big O vs. Big Theta
- Big O is used when you need to determine the worst-case scenario, such as ensuring your application can handle peak traffic without performance degradation. For example, think about a gaming application where thousands of players might attempt to access the game simultaneously during a beta release. In this case, you’d focus on Big O to ensure the game can handle that load without crashing.
- Big Theta, on the other hand, is used when you want to analyze the average performance of your algorithm. If you’re working on a web search engine, for instance, you would care about the typical response time for the majority of users, rather than the worst-case scenario where everything slows down.
Big O, Big Theta, and Big Omega Summary
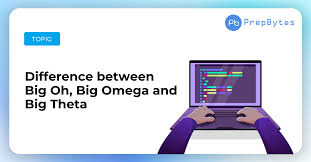
To make these notations easier to remember, think of the following mnemonic:
- Big O is the worst-case scenario: “Woah, woah, worst case, and O has one side.”
- Big Theta is the average-case scenario: “The typical, two-sided case (Θ)!”
- Big Omega is the best-case scenario: “One-sided utopia, Omega is the ultimate best!”
Real-World Examples of Big O, Big Theta, and Big Omega
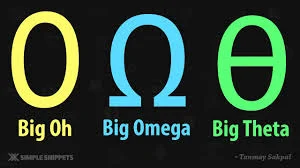
Sorting Algorithms:
- Bubble Sort: In the worst case (Big O), bubble sort has a time complexity of O(n²) because each element needs to be compared with every other element.
- Merge Sort: Merge sort has a time complexity of O(n log n) in the worst case, which is significantly better than bubble sort.
- Quick Sort: In the best case (Big Omega), quicksort has a time complexity of Ω(n log n), but in the worst case, it can degrade to O(n²).
Searching Algorithms:
- Binary Search: In a sorted array, binary search has a time complexity of O(log n) in the worst case (Big O) and Ω(log n) in the best case, as it halves the search space at each step.
- Linear Search: In the worst case, linear search will check every element, resulting in O(n), but in the best case (Big Omega), it might find the target on the first try.
Conclusion: Optimizing Algorithms with Big O, Big Theta, and Big Omega
Understanding the difference between Big O, Big Theta, and Big Omega is critical for optimizing algorithms in real-world applications. Each notation provides valuable insights into how an algorithm performs under different conditions—whether it’s the worst-case, average-case, or best-case scenario. By using these notations, you can assess the efficiency of your code and make informed decisions about how to optimize your algorithms for performance.
The key takeaway is that Big O is used to guarantee that your algorithm will not exceed a certain time limit in the worst-case scenario, Big Theta is helpful for understanding how your algorithm performs on average, and Big Omega gives you the ideal, best-case performance. All three notations work together to give a holistic view of your algorithm’s efficiency, enabling you to build fast, reliable, and scalable systems.