Machine learning (ML) is a powerful tool for predictive analytics, but optimizing ML models often requires significant experimentation. The most time-consuming part of this process is hyperparameter tuning—manually adjusting hyperparameters (such as learning rates or number of hidden layers) to optimize the model’s performance. In this context, Katib, an open-source tool integrated with Kubeflow, automates hyperparameter optimization (HPO) by systematically searching for the best set of hyperparameters.
This blog will walk you through the process of setting up Katib for automated hyperparameter tuning using Docker, Vagrant, and Kubernetes. By automating the hyperparameter tuning process, you can improve the efficiency of your ML workflows and achieve better performance with less manual intervention.
What is Katib?
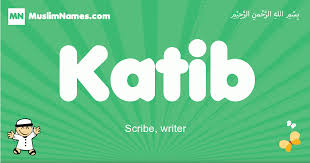
Katib is an open-source, automated machine learning (AutoML) tool designed to optimize hyperparameters by integrating with Kubernetes and Kubeflow. Katib supports various optimization algorithms, including Random Search, Grid Search, Bayesian Optimization, and others, to help you find the best set of hyperparameters for your machine learning models.
By leveraging Kubernetes and Docker, Katib provides a scalable and easy-to-use framework for hyperparameter optimization. It automates the often manual and error-prone process of tuning hyperparameters, making it easier for data scientists to experiment with and optimize machine learning models.
Why Automate Hyperparameter Tuning?
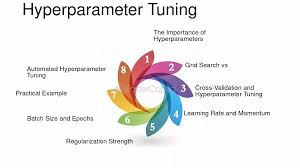
Manual hyperparameter tuning is time-consuming and inefficient. The hyperparameter search space grows exponentially as the number of hyperparameters increases, making it impossible to manually explore all possible combinations. This challenge is further compounded by:
- Not transferable: Hyperparameters optimized for one dataset or model often don’t work well for others.
- Inefficient: Manually adjusting hyperparameters can lead to wasted time and resources.
- Tracking issues: Keeping track of experiments and their corresponding metrics becomes difficult without automation.
Automating the hyperparameter tuning process can save time, reduce human error, and find the best-performing hyperparameters much faster. Katib addresses these challenges by providing a streamlined solution for hyperparameter optimization that scales with your needs.
How to Set Up Katib Locally Using Docker, Vagrant, and Kubernetes
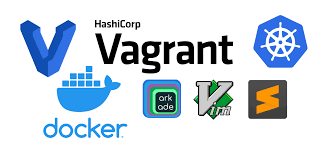
In this tutorial, we will install and run Katib using Docker and Kubernetes locally. This will allow you to quickly test and experiment with Katib’s capabilities before scaling to more extensive cloud environments.
Step 1: Install Prerequisites
Before we dive into setting up Katib, ensure that you have the following tools installed:
- Docker: Install Docker Desktop to manage containers. Docker is necessary for running the Kubernetes cluster locally.
- Vagrant: Install Vagrant to set up a virtual machine environment on your local machine. Vagrant will help you deploy a local Kubernetes environment using Minikube or any other supported Kubernetes tool.
- Minikube: Minikube helps you create a local Kubernetes cluster, making it ideal for testing Kubernetes workloads without needing cloud resources.
- kubectl: This is the command-line tool for interacting with your Kubernetes cluster. You can install it by following the instructions here.
Step 2: Set Up Kubernetes with Minikube
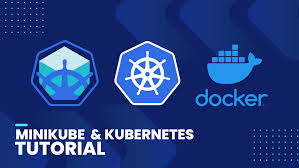
Once the prerequisites are installed, the next step is to set up a local Kubernetes cluster. Here’s how to do it:
- Start Minikube to create a local Kubernetes cluster:bashCopy
minikube start
- Set up
kubectl
to use the local Minikube cluster:bashCopykubectl config use-context minikube
- Verify that your Kubernetes cluster is running by checking the cluster nodes:bashCopy
kubectl get nodes
Step 3: Install Katib
Now that your Kubernetes cluster is set up, we can proceed with installing Katib.
- Install the Katib control plane by applying the following YAML configuration:bashCopy
kubectl apply -k "github.com/kubeflow/katib.git/manifests/v1beta1/installs/katib-standalone?ref=master"
- Verify the installation by checking the status of the Katib components:bashCopy
kubectl get pods -n kubeflow
You should see thekatib-controller
,katib-db-manager
,katib-mysql
, andkatib-ui
components running.
Step 4: Run an Example Hyperparameter Optimization Experiment
Katib supports various optimization algorithms, such as Random Search and Bayesian Optimization. To test Katib, we’ll run a simple Random Search experiment to optimize hyperparameters for an ML model.
- Download an example Random Search experiment YAML file:bashCopy
curl https://raw.githubusercontent.com/kubeflow/katib/master/examples/v1beta1/hp-tuning/random.yaml --output random.yaml
- Modify the YAML file to specify the correct namespace for your setup. Replace
kubeflow-user-example-com
with your namespace:yamlCopynamespace: kubeflow-user-example-com
- Apply the YAML file to create the experiment:bashCopy
kubectl apply -f random.yaml
- Monitor the experiment progress:bashCopy
kubectl -n kubeflow-user-example-com get experiment random -o yaml
Step 5: Access the Katib UI
Katib also provides a web UI for visualizing the progress of experiments and analyzing the results. To access the Katib UI:
- Set up port forwarding to access the Katib UI on your local machine:bashCopy
kubectl port-forward svc/katib-ui -n kubeflow 8080:80
- Open the Katib UI in your browser at http://localhost:8080/katib/.
In the UI, you can monitor the experiment’s progress, view metrics for each trial, and identify the best hyperparameters for your model.
Step 6: Scaling to Cloud
Once you’ve tested Katib locally, you can scale your hyperparameter optimization workflows to the cloud using AWS, Google Cloud, or Azure. Kubernetes and Kubeflow provide the necessary infrastructure for deploying Katib in production environments, where you can perform large-scale optimization tasks across multiple nodes.
Conclusion
Katib simplifies the process of hyperparameter optimization, making it easier to find the best set of hyperparameters for your machine learning models. By automating the tuning process, Katib reduces the time and resources spent on manual adjustments and increases the accuracy of your models. Whether you’re working locally with Docker and Kubernetes or scaling to the cloud, Katib provides a flexible solution for optimizing your machine learning workflows.
With tools like Vagrant, VirtualBox, and Minikube, you can easily set up Katib on your local machine to test and experiment with hyperparameter optimization before deploying in a production environment. Embrace the power of automated machine learning with Katib, and optimize your models for better performance, faster!