comprehensive guide to Data Labeling: The Backbone of Supervised Machine Learning 2024
In the realm of machine learning, particularly in supervised learning, data labeling is a crucial process. Labels transform raw data into structured inputs, enabling models to learn patterns and make predictions. However, acquiring high-quality labeled data is often challenging, expensive, and time-intensive.
This blog explores the importance of data labeling, common challenges, and innovative techniques like weak supervision, semi-supervision, transfer learning, and active learning to address labeling challenges.
Why Data Labeling is Important
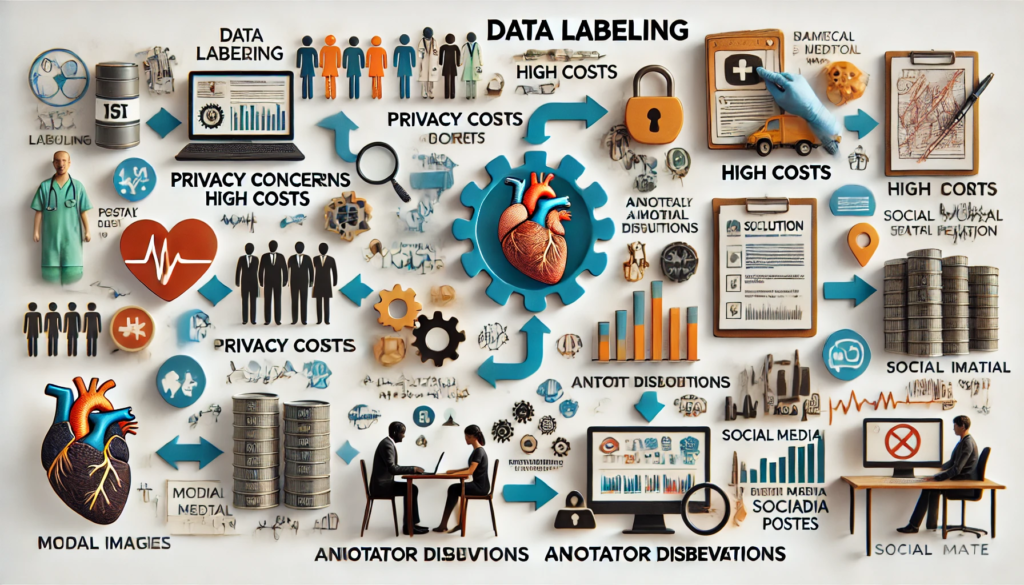
Most machine learning models rely on labeled data to learn relationships between inputs and outputs. The quality and quantity of labeled data directly impact the performance of these models.
Examples of Labeled Data:
- Click-through Rates:
- Labels indicate whether a user clicked on an ad.
- Recommendation Systems:
- Labels represent user interactions, such as clicks or purchases.
- Medical Imaging:
- Labels classify scans as “normal” or “abnormal.”
While some tasks have natural labels (e.g., clicks), others require manual labeling, which introduces unique challenges.
Challenges in Data Labeling
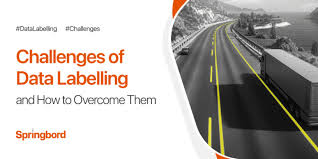
- Expense:
- Hand-labeling data, especially when expertise is required (e.g., radiologists for X-rays), can be costly.
- Privacy Concerns:
- Labeling sensitive data like patient records may require on-premise annotation, increasing complexity.
- Time-Intensive:
- Annotating complex datasets, like speech transcriptions, can be slow, hindering model iterations.
- Label Multiplicity:
- Multiple annotators with varying expertise often produce conflicting labels, complicating the training process.
The Label Multiplicity Problem
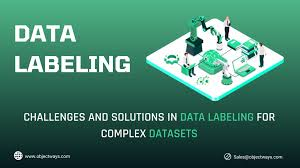
When data is labeled by multiple annotators, disagreements are common, especially for tasks requiring high domain expertise. For example:
- In an entity recognition task, one annotator may identify “Galactic Emperor,” while another chooses “First Galactic Empire.”
Solutions to Minimize Disagreement:
- Clear Problem Definitions:
- Specify detailed guidelines for annotators.
- Consensus Building:
- Combine annotations from multiple sources and resolve conflicts.
- Data Lineage:
- Track the origin of each label to identify and debug biases.
Techniques to Address Labeling Challenges
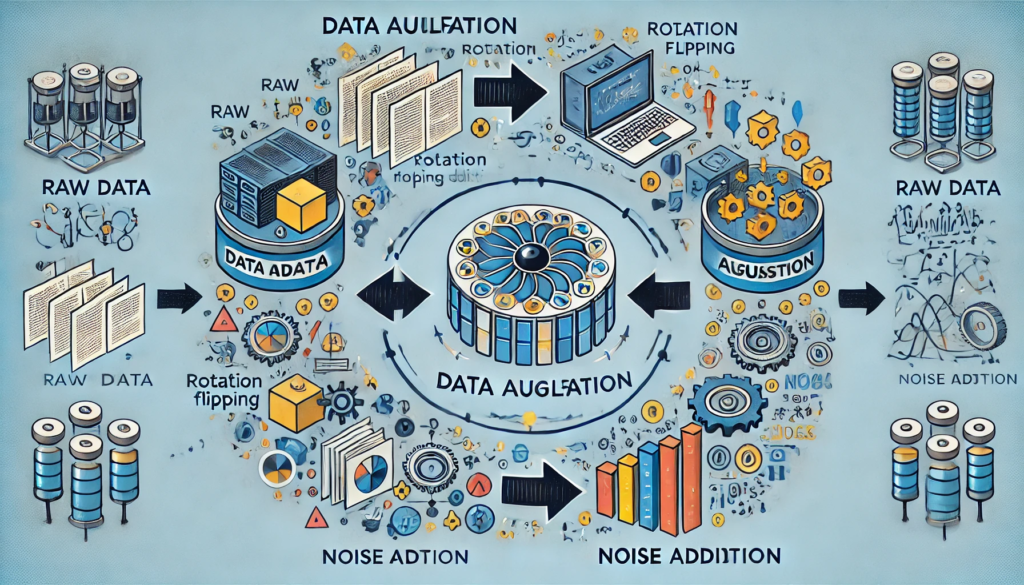
1. Weak Supervision
Definition: Uses heuristics or external data sources to generate labels programmatically, reducing the reliance on manual labeling.
- Example:pythonCopyEdit
def labeling_function(note): if "pneumonia" in note: return "EMERGENT"
- Advantages:
- Scales quickly by applying labeling functions (LFs) across datasets.
- Encodes subject-matter expertise for reusable heuristics.
- Challenges:
- Labels may be noisy, requiring denoising techniques.
2. Semi-Supervised Learning
Definition: Leverages a small labeled dataset to generate labels for a larger unlabeled dataset.
Common Methods:
- Self-Training:
- Train a model on labeled data, use it to predict labels for unlabeled samples, and retrain iteratively.
- Clustering-Based:
- Group similar data points and assign labels to clusters.
Advantages:
- Cost-effective for large datasets with limited labels.
- Incorporates unlabeled data to improve performance.
3. Transfer Learning
Definition: Utilizes pre-trained models from one task as a starting point for another task.
Key Steps:
- Train a base model (e.g., language modeling) on abundant, unlabeled data.
- Fine-tune the model on a smaller labeled dataset for the target task.
Benefits:
- Reduces the need for extensive labeled data.
- Widely used in NLP (e.g., BERT) and computer vision (e.g., ImageNet).
4. Active Learning
Definition: Allows the model to select the most informative data points for labeling by human annotators.
Heuristics for Selection:
- Uncertainty Sampling:
- Choose samples with the lowest confidence scores.
- Query by Committee:
- Use a “committee” of models to identify samples with the highest disagreement.
Advantages:
- Maximizes the efficiency of labeling efforts.
- Focuses on samples that improve model performance the most.
Best Practices for Data Labeling
- Prioritize Quality Over Quantity:
- A small, high-quality labeled dataset often outperforms a large, noisy dataset.
- Use Data Lineage:
- Track the origin of data and labels to identify biases and errors.
- Combine Human and Machine Efforts:
- Use weak supervision or transfer learning to augment manual efforts.
- Monitor Label Distribution:
- Ensure balanced labels to avoid class imbalances in the dataset.
Conclusion
Data labeling is foundational to supervised machine learning but comes with significant challenges. By combining manual efforts with techniques like weak supervision, semi-supervision, transfer learning, and active learning, organizations can efficiently generate high-quality labeled datasets.
Ready to optimize your data labeling process? Start implementing these techniques today to build robust machine learning models!