comprehensive guide to Engineering Good Features: Building Robust Machine Learning Models 2024
In the journey of building machine learning models, features serve as the backbone, transforming raw data into actionable inputs. While adding more features can often lead to better model performance, this isn’t always the case. Understanding how to evaluate, select, and engineer good features is essential for creating efficient, generalizable, and impactful models.
This blog explores the importance of feature engineering, the challenges of having too many features, and key strategies for measuring and ensuring feature quality.
The Importance of Good Features
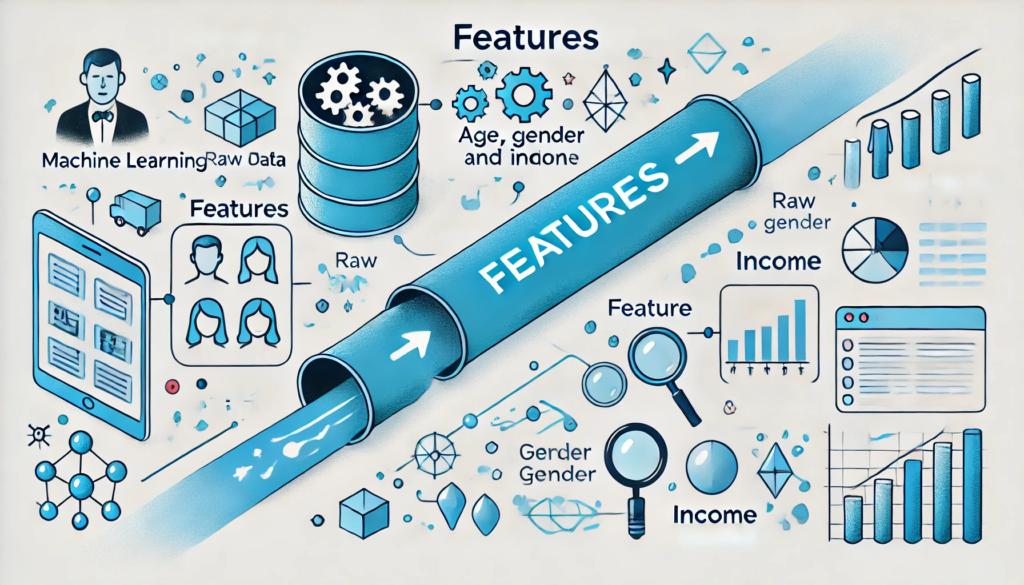
Features play a pivotal role in determining a machine learning model’s success. Generally, adding features increases model performance by offering more descriptive data. However, the growth in the number of features must be managed carefully to avoid pitfalls like:
- Data Leakage:
- Features may inadvertently expose information about the target variable.
- Overfitting:
- Too many features can make the model overly complex, reducing generalization to unseen data.
- Increased Latency:
- Serving models with many features can lead to slower predictions and higher computational costs.
Two Key Metrics for Evaluating Features:
- Feature Importance:
- Measures the impact of a feature on model performance.
- Feature Generalization:
- Assesses how well a feature applies to unseen data.
Too Many Features: The Hidden Costs
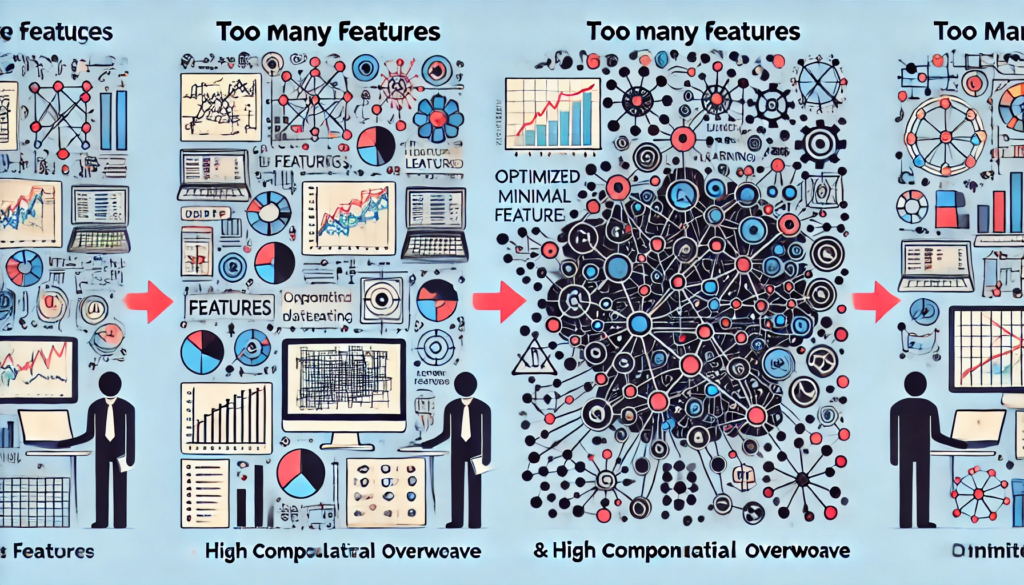
While adding more features seems appealing, it comes with significant challenges:
- Memory Overhead:
- More features require more memory, which may lead to the need for expensive infrastructure.
- Inference Latency:
- Online predictions become slower, especially if features require preprocessing.
- Technical Debt:
- Useless features can complicate data pipelines, requiring maintenance even when they don’t contribute to the model.
Feature Importance
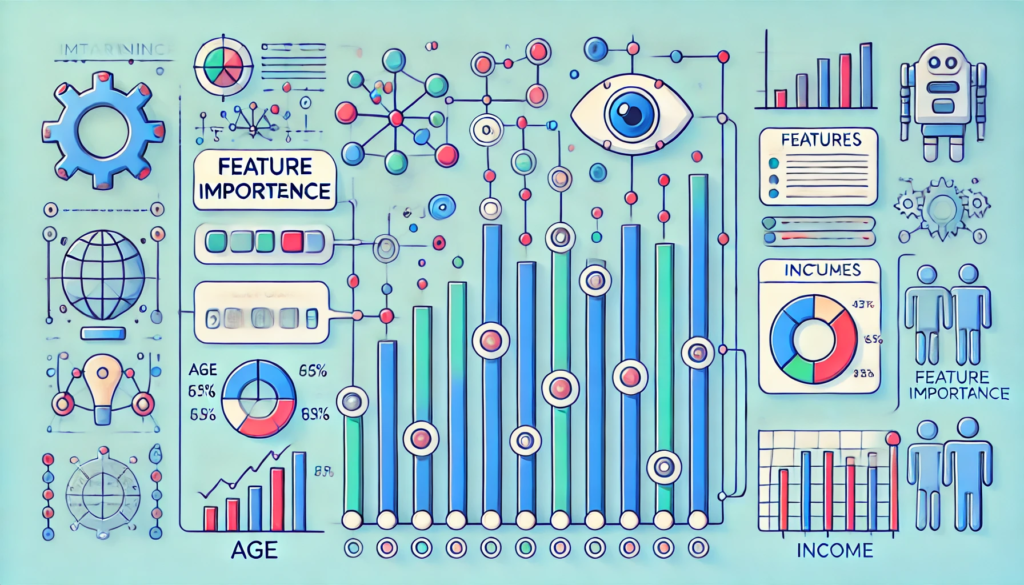
How to Measure Feature Importance:
- Built-in Methods:
- Algorithms like XGBoost provide feature importance as part of the training process.
- SHAP (SHapley Additive Explanations):
- A model-agnostic method that explains feature contributions to individual predictions.
- InterpretML:
- Tools for evaluating the overall impact of features on models.
Key Insights:
- A small subset of features often accounts for the majority of a model’s performance.
- Example: In a click-through rate prediction model by Facebook, the top 10 features contributed to half the model’s feature importance, while the bottom 300 features had minimal impact.
Feature Generalization
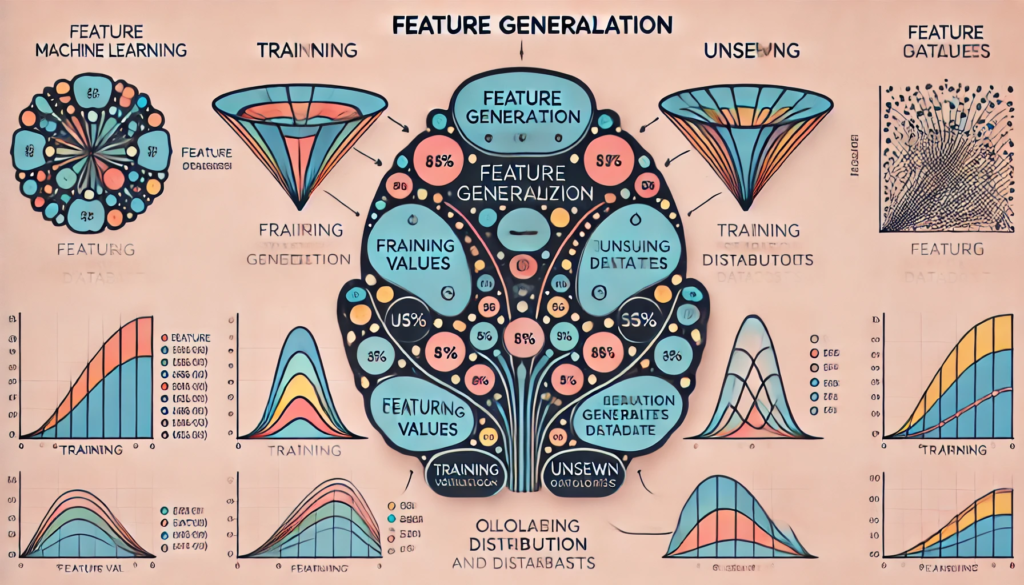
Why Generalization Matters:
A feature’s value lies in its ability to help the model make accurate predictions on unseen data. Features that don’t generalize well can hurt model performance.
Two Aspects of Generalization:
- Feature Coverage:
- Measures the percentage of samples in which a feature is present.
- Example:
- A feature available in only 1% of the dataset likely won’t generalize well.
- Distribution of Feature Values:
- Features with mismatched distributions between training and test datasets may lead to poor generalization.
- Example:
- A model trained with
DAY_OF_WEEK
values from Monday to Saturday but tested with Sunday may fail to generalize.
- A model trained with
Specificity vs. Generalization:
- Balancing specificity and generalization is crucial.
- Example:
IS_RUSH_HOUR
(generalizable) may lack the detail provided byHOUR_OF_DAY
(specific), but combining them may offer the best of both worlds.
Feature Engineering Best Practices
- Evaluate Features Early:
- Use statistical and domain knowledge to assess features during development.
- Store and Reuse Features:
- Leverage feature stores to standardize and share features across teams.
- Remove Useless Features:
- Regularly prune features with low importance or poor generalization.
- Version Features:
- Maintain a version history to track changes and revert if needed.
- Continuously Monitor Features:
- Evaluate feature performance over time to ensure relevance.
Challenges in Feature Engineering
- Handling Missing Data:
- Missing values reduce feature coverage and impact model reliability.
- Maintaining Consistency:
- Ensure feature definitions remain stable across training and inference pipelines.
- Avoiding Leakage:
- Features that expose future or target information can lead to misleadingly high performance during training but fail in production.
Tools for Feature Evaluation and Engineering
- SHAP:
- Explains feature contributions and importance at the model and prediction levels.
- Feature Stores:
- Central repositories for storing and managing feature definitions and values.
- Feature Selection Algorithms:
- Tools like Recursive Feature Elimination (RFE) or mutual information for selecting the most impactful features.
Conclusion
Good feature engineering is the backbone of successful machine learning models. By understanding the principles of feature importance and generalization, practitioners can ensure their models are efficient, reliable, and effective. While more features may seem advantageous, prioritizing high-quality, generalizable features ultimately leads to better outcomes and reduced complexity.
Ready to elevate your machine learning models with well-engineered features? Start optimizing your features today!