comprehensive guide to Feature Systems in Machine Learning: Managing the Flow from Data to Features 2024
In machine learning (ML), features are the cornerstone of building effective models. However, creating, managing, and evaluating features for complex ML systems can be daunting. Feature systems provide a structured approach to managing the flow of data, turning raw inputs into actionable features used during training and inference.
This blog explores the components of feature systems, including data ingestion systems, feature stores, and feature quality evaluation, highlighting their importance in modern ML workflows.
What is a Feature System?
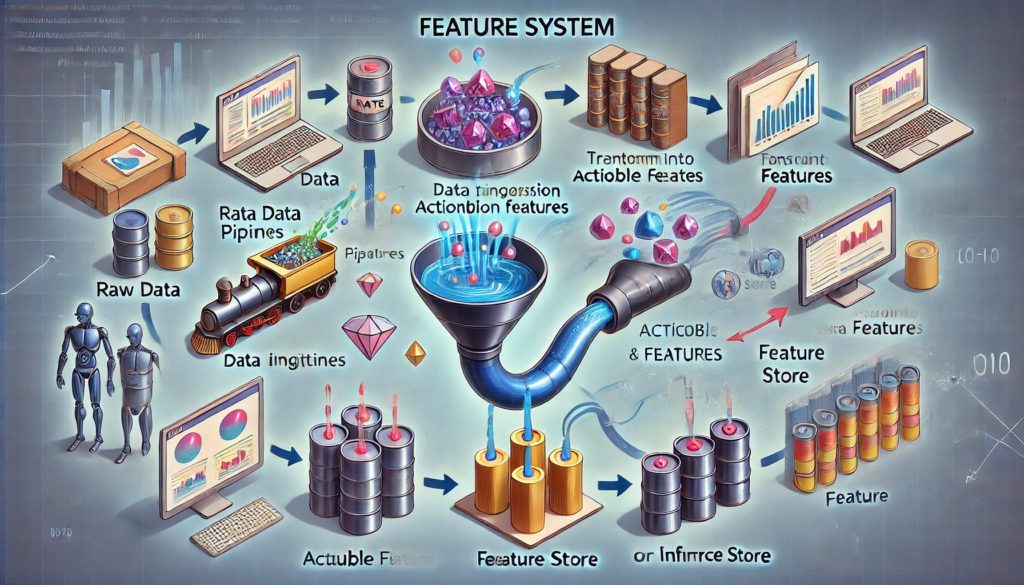
A feature system is a set of subsystems designed to manage the flow of data from raw inputs to ready-to-use features for ML models. It ensures:
- Consistency: Unified management of feature definitions and values.
- Efficiency: Reusability and on-demand access to features.
- Reliability: Robust pipelines for data ingestion and feature extraction.
Key Components:
- Data Ingestion System:
- Reads raw data, extracts features, and stores them in a feature store.
- Feature Store:
- A centralized storage system for extracted feature values and definitions.
- Feature Quality Evaluation System:
- Measures the impact of features on model performance.
Data Ingestion Systems
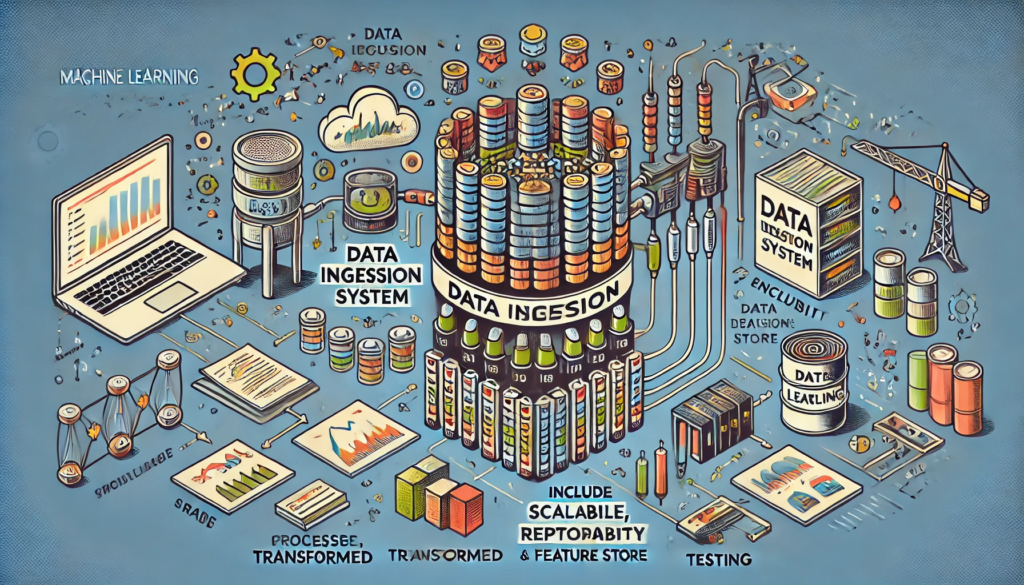
The data ingestion system is responsible for reading raw data, applying feature extraction code, and storing the resulting feature values in a feature store.
Key Requirements:
- Scalability:
- Must handle large-scale data extraction tasks efficiently.
- Repeatability:
- Should function as a repeatable, monitored pipeline.
- Reliability:
- Provides tools for feature authors to write reliable extraction code.
Best Practices:
- Versioning:
- Features should be versioned to track changes and prevent unintended consequences.
- Testing:
- Include systems to test feature-extraction code for correctness.
- Staging Environment:
- Allow feature authors to test features on small datasets before full-scale deployment.
Feature Stores
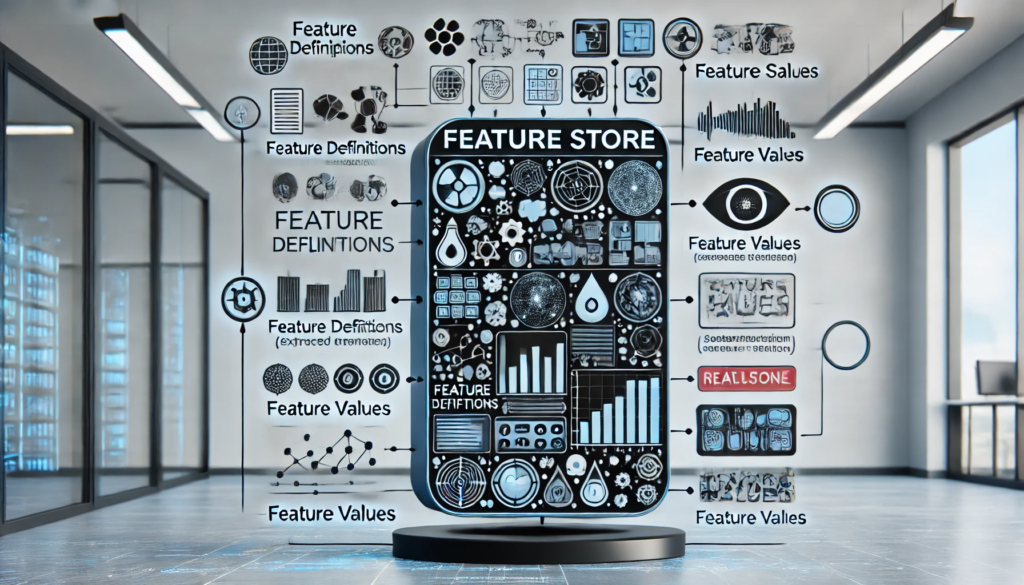
A feature store is a centralized repository for storing and managing extracted feature values. It plays a critical role in modern ML systems by ensuring consistency and efficiency during training and inference.
Core Functions of a Feature Store:
- Storing Feature Definitions:
- Stores code for feature extraction alongside metadata.
- Serving Feature Values:
- Provides fast, consistent access to features during training and inference.
- Metadata Management:
- Coordinates metadata about features for better usability and traceability.
Key Questions to Consider:
- Data Access Patterns:
- Is the data read frequently or only during training?
- Storage Type:
- Should the data be stored as columns (structured) or blobs (unstructured)?
- Privacy and Security:
- Are there any special privacy requirements for the stored data?
Feature Quality Evaluation Systems
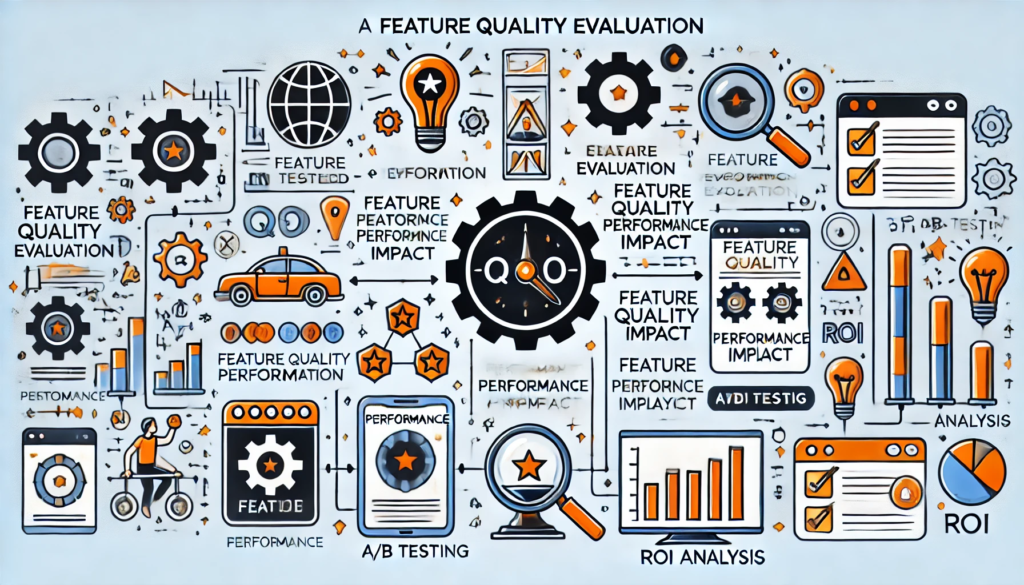
As new features are developed, it’s essential to evaluate their contribution to model performance. A feature quality evaluation system ensures that only impactful features are integrated into the model.
Evaluation Techniques:
- A/B Testing:
- Compare the performance of models with and without the new feature.
- Retraining:
- Train the model with a single additional feature to evaluate its impact.
- Return on Investment (ROI):
- Calculate the cost-benefit ratio of adding a new feature.
Challenges in Building Feature Systems
- Scalability:
- Handling large-scale data and feature extraction tasks efficiently.
- Consistency:
- Ensuring consistent feature values across training and inference workflows.
- Testing and Validation:
- Developing robust testing frameworks for feature-extraction code.
- Resource Management:
- Balancing the cost of storing and processing features with their value.
Future Trends in Feature Systems
- Automated Feature Engineering:
- Leveraging AI to automate feature extraction and evaluation.
- Real-Time Feature Serving:
- Providing real-time access to features for dynamic use cases like fraud detection.
- Cloud-Native Feature Stores:
- Adopting serverless architectures for scalability and cost-efficiency.
Conclusion
Feature systems are critical to managing the complex flow of data in modern ML pipelines. From data ingestion and feature storage to quality evaluation, these systems ensure that machine learning models are built on a foundation of reliable, efficient, and impactful features.
By leveraging feature systems, organizations can enhance their ML workflows, improve model performance, and scale analytics efforts effectively.
Are you ready to optimize your machine learning pipelines with robust feature systems? Start building smarter workflows today!