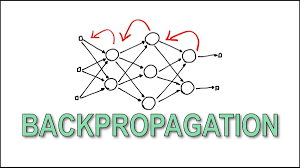
comprehensive guide to Gradient Descent and Backpropagation for Neural Network Training 2024
Training deep learning models can often seem like a daunting task, especially with large datasets and complex architectures. However, at the core of many successful models lies a set of powerful optimization techniques: Gradient Descent and Backpropagation. These two algorithms work together to adjust the parameters of a model, minimizing the error between the model’s predictions and the actual outcomes.
In this blog, we will explore the essentials of Gradient Descent and Backpropagation, how they work together in training neural networks, and some of the key concepts around efficiency, step sizes, and optimization techniques.
What is Gradient Descent?
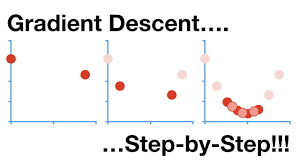
Gradient Descent (GD) is an iterative optimization algorithm used to minimize the loss function of a neural network. The core idea is to update the model’s parameters (like weights and biases) in the direction that reduces the loss function, which is a measure of how far the model’s predictions are from the actual values.
The algorithm works by computing the gradient of the loss function concerning each parameter, indicating the direction of the steepest descent. In other words, it guides the model to adjust its parameters toward a configuration that minimizes the loss.
The Process of Gradient Descent:
- Initialization: The model parameters (weights and biases) are initialized with random values.
- Gradient Calculation: The gradient (or derivative) of the loss function with respect to each parameter is calculated.
- Update Parameters: The parameters are updated by moving in the direction opposite to the gradient, ensuring that the loss function is minimized.
- Repeat: This process repeats iteratively until the loss function reaches a minimum (or converges to an acceptable level).
This iterative process is performed for a number of epochs, each consisting of forward propagation, loss evaluation, and backpropagation.
What is Backpropagation?
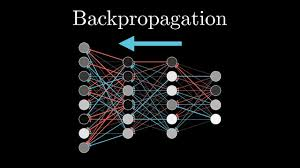
Backpropagation is a technique used to efficiently calculate the gradients of the loss function with respect to each parameter in a neural network. It involves propagating the error backward through the network, layer by layer, using the chain rule of calculus. This allows for the efficient computation of the gradients required for gradient descent.
How Backpropagation Works:
- Forward Pass: The network computes predictions based on the input data.
- Loss Calculation: The loss (or error) is computed by comparing the predicted output to the true labels.
- Backward Pass: Backpropagation starts from the output layer and propagates the error backward through the network, computing the gradients for each parameter.
- Gradient Update: Using the computed gradients, the parameters are updated to minimize the loss function.
Backpropagation is essential because it allows us to compute the gradients for all parameters in the network, ensuring that gradient descent can effectively update them.
The Interaction Between Gradient Descent and Backpropagation
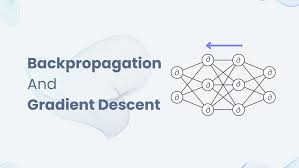
During the training process, forward propagation computes the predicted output from the input data. Then, backpropagation calculates the gradients of the loss function concerning the network’s parameters. Finally, gradient descent uses these gradients to update the parameters iteratively.
The entire process works together as follows:
- Forward Propagation: Computes the output.
- Loss Evaluation: Calculates how far off the model’s prediction is from the actual labels.
- Backpropagation: Computes the gradients of the loss with respect to the model parameters.
- Gradient Descent: Updates the parameters in the direction that reduces the loss.
This cycle is repeated for each batch of data, and over multiple epochs, the model parameters are optimized.
Step Size: A Critical Factor in Gradient Descent
One of the most crucial elements of gradient descent is the step size (also called the learning rate). This value determines how large a step the algorithm should take in the direction of the gradient during each iteration.
- A large step size might result in overshooting the optimal point, causing the model to diverge.
- A small step size may lead to a slow convergence, making the training process take much longer than necessary.
Thus, finding an appropriate step size is vital for the efficiency of gradient descent.
Line Search and Backtracking Line Search
To deal with finding an appropriate step size, line search and backtracking line search are common techniques. These methods iteratively adjust the step size to find the optimal value that decreases the loss function sufficiently.
Convex Functions and Gradient Descent
Gradient descent works particularly well for convex functions, where the global minimum is the only minimum, and local optima do not exist. If a function is convex, any critical point found by gradient descent is guaranteed to be the global minimum.
However, not all functions encountered in deep learning are convex. For example, deep neural networks often involve non-convex loss functions, which means that gradient descent may converge to local minima or saddle points instead of the global minimum.
Subgradient Descent: Handling Non-Differentiable Functions
In the case of non-differentiable convex functions, subgradients are used instead of gradients. A subgradient is a generalization of the gradient, allowing gradient descent to handle functions that are not smooth or differentiable at all points.
Subgradient descent is particularly useful for models where the loss function involves non-differentiable points or discontinuities. While the concept of subgradients is more advanced, it allows gradient descent to operate effectively in a broader range of optimization problems.
Theoretical Guarantees and Convergence
When using gradient descent, especially in cases involving subgradients or non-convex functions, certain theoretical guarantees can help us understand the algorithm’s behavior:
- Diminishing Step Size: For guaranteed convergence, the step size typically needs to diminish over time, following specific rules to ensure that the algorithm doesn’t jump too far or get stuck.
- Convergence Criteria: Convergence is achieved when the loss function reaches a sufficiently small value, or when the changes in parameters become insignificant between epochs.
Conclusion: Gradient Descent as the Backbone of Neural Network Training
Gradient descent and backpropagation are foundational algorithms in the training of neural networks. Gradient descent helps minimize the loss function by adjusting the model’s parameters, while backpropagation efficiently calculates the gradients needed for these adjustments.
By understanding how these algorithms work together, how to manage step sizes, and how to handle non-convex functions, you can train more effective and efficient models. While gradient descent offers great promise for optimizing neural networks, understanding its nuances, such as subgradients and step size rules, ensures that you can harness its full potential.
Whether you’re working on deep learning or other optimization problems, gradient descent and backpropagation are indispensable tools in the data scientist’s toolkit.