comprehensive guide to Machine Learning Metadata Store: Centralizing Model Management 2024
As machine learning systems grow in complexity, managing metadata becomes critical for ensuring efficiency, traceability, and performance. Machine Learning (ML) Metadata Stores are purpose-built platforms that centralize and organize metadata generated during the lifecycle of ML models.
This blog explores the concept of ML metadata, the role of metadata stores, their importance in machine learning pipelines, and how to set up an effective metadata management system.
Why is Metadata Important in ML?
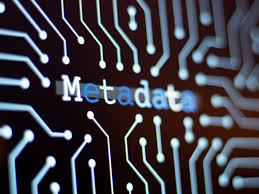
Metadata provides context and traceability for every aspect of an ML system, answering critical questions like:
- How was the model built?
- Which dataset and parameters were used?
- What were the evaluation results and metrics?
- Who is responsible for maintaining this model?
Without metadata, managing experiments, debugging, retraining, and ensuring compliance becomes cumbersome, especially as ML systems scale.
Key Types of Metadata in Machine Learning
1. Experiment Metadata
Captures information about model training runs, such as:
- Data Version: Reference to the dataset and its MD5 hash.
- Environment Configuration: Dockerfile,
requirements.txt
, or Conda files to recreate the training environment. - Code Version: Git SHA or a snapshot of the code used for training.
- Hyperparameters: Configuration of model training parameters.
- Training Metrics: Losses and evaluation metrics like accuracy and F1-score.
2. Artifact Metadata
Represents inputs and outputs of ML pipelines, such as datasets, model weights, and predictions. Key attributes include:
- References: Paths to the dataset or model in storage (e.g., S3 bucket, file system).
- Versions: MD5 hashes to track changes over time.
- Previews: Snapshots or column names for tabular data.
3. Model Metadata
Focuses on the trained models themselves:
- Model Package: Binary or location of the model asset.
- Model Version: Versions of code, dataset, and hyperparameters used.
- Evaluation Records: History of validation results and metrics.
- Drift Metrics: Measures like data and concept drift for production models.
4. Pipeline Metadata
Tracks the lifecycle of automated pipelines:
- Input/Output Steps: Information about the dependencies and outputs of each step.
- Cached Outputs: Intermediate results for resuming workflows efficiently.
- Trigger Events: Details about the events (e.g., performance drops, new data arrival) that initiate pipeline runs.
What is an ML Metadata Store?
An ML Metadata Store is a centralized repository that:
- Logs and Stores:
- Captures and stores all metadata related to experiments, models, artifacts, and pipelines.
- Monitors and Queries:
- Enables filtering, querying, and comparing metadata across models and experiments.
- Organizes and Displays:
- Provides a user-friendly interface for exploring model-related information.
Key Features:
- API or SDK Integration:
- Tools like MLflow, Neptune, or TensorFlow Metadata (TFMD) provide APIs for seamless logging.
- Versioning:
- Tracks changes to datasets, models, and experiments.
- Collaboration:
- Facilitates team workflows by maintaining shared metadata.
Metadata Repository vs. Registry vs. Store
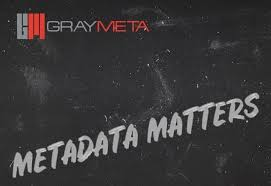
- Metadata Repository:
- Stores metadata objects and their relationships.
- Example: Logging evaluation metrics in GitHub or an experiment tracking tool.
- Metadata Registry:
- Used to register important metadata checkpoints for easy access later.
- Example: A model registry listing production-ready models.
- Metadata Store:
- A comprehensive platform that integrates repositories and registries, enabling end-to-end metadata management.
Setting Up an ML Metadata Management System
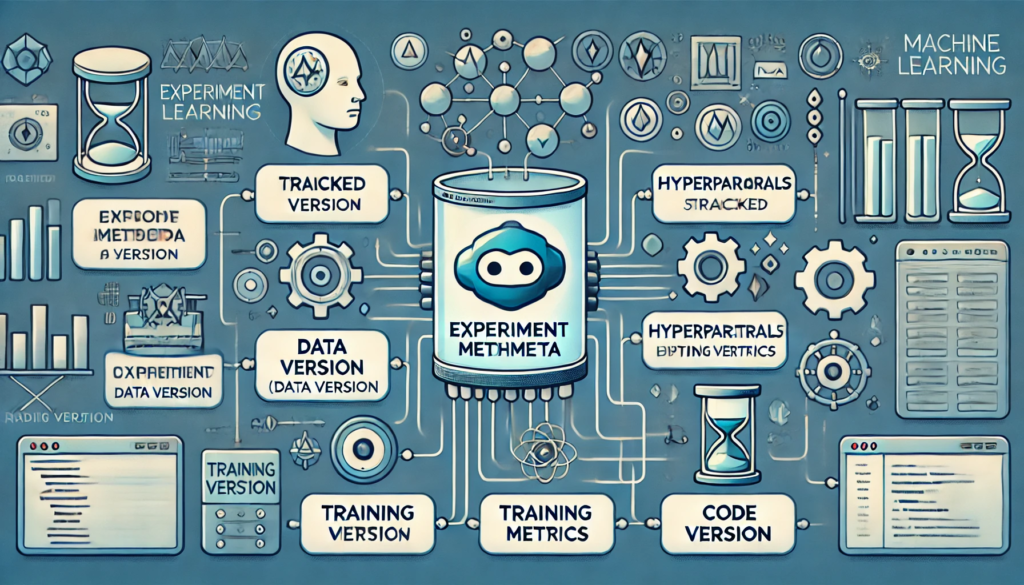
1. Pipeline-First Approach
Focuses on automating and orchestrating model pipelines:
- Example Tools: Kubeflow, TensorFlow Extended (TFX).
- Ideal For: Organizations prioritizing CI/CD pipelines and large-scale automation.
2. Model-First Approach
Centers around managing and monitoring trained models:
- Example Tools: MLflow, Neptune.
- Ideal For: Teams optimizing experiments and deployed model performance.
Build vs. Maintain vs. Buy
- Build a System Yourself:
- Custom solutions tailored to specific needs.
- Challenges: High maintenance and development costs.
- Maintain Open Source:
- Use platforms like MLflow or TFX.
- Advantages: Cost-effective, with community support.
- Buy a Solution:
- Enterprise tools like Databricks MLflow or AWS SageMaker Model Registry.
- Advantages: Ready-to-use with enterprise-grade features.
Benefits of ML Metadata Stores
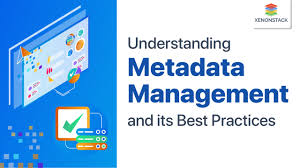
- Traceability:
- Ensures accountability for datasets, features, and model versions.
- Debugging:
- Simplifies identifying errors in pipelines and experiments.
- Compliance:
- Tracks lineage for regulatory and audit purposes.
- Collaboration:
- Enables teams to share, review, and iterate on experiments efficiently.
Future Trends in Metadata Management
- Real-Time Metadata Updates:
- Systems that update metadata dynamically during production runs.
- AI-Assisted Metadata:
- Using AI to recommend configurations or highlight anomalies.
- Cloud-Native Metadata Systems:
- Serverless architectures for scalable and cost-efficient metadata storage.
Conclusion
An ML Metadata Store is an indispensable tool for managing modern machine learning workflows. By centralizing metadata, teams can streamline operations, enhance model reliability, and build systems that scale seamlessly. Whether you build, adopt open-source, or buy a solution, investing in metadata management will pay dividends in efficiency and accuracy.
Ready to transform your ML workflow with metadata management? Start building smarter systems today!