comprehensive guide to ML Experiment Tracking: A Blueprint for Reproducible Machine Learning 2024
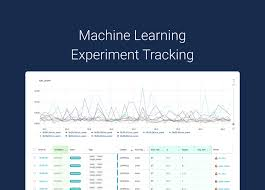
Developing machine learning models often involves running numerous experiments with varying configurations, data versions, and hyperparameters. Without a system to track these experiments, managing them can quickly become chaotic. ML Experiment Tracking provides a structured way to capture, organize, and analyze all relevant metadata from experiments, ensuring reproducibility and efficiency.
This blog explores the concept of ML experiment tracking, its importance, and practical approaches to implement it.
What is ML Experiment Tracking?
Experiment tracking is the process of saving all relevant information about machine learning experiments, such as:
- Training scripts.
- Environment configurations.
- Data versions.
- Hyperparameter settings.
- Evaluation metrics.
- Model artifacts and predictions.
This metadata ensures that every experiment is reproducible and easy to analyze.
Why Does Experiment Tracking Matter?
- Organized Experimentation:
- Without a tracking system, experiment results are often scattered across devices, notebooks, or cloud instances.
- Centralized tracking keeps all experiment metadata in one place.
- Reproducibility:
- Ensures you can recreate any experiment by logging code, data, and configurations.
- Comparative Analysis:
- Facilitates quick comparison of parameters, metrics, and performance across experiments.
- Collaboration:
- Enables teams to share experiment results, boosting transparency and productivity.
Key Components of an Experiment Tracking System
1. Experiment Database
A centralized repository for storing experiment metadata. It allows for:
- Logging: Recording metadata such as hyperparameters and metrics.
- Querying: Searching for specific experiments or configurations.
2. Experiment Dashboard
A visual interface that provides insights into experiments. Common features include:
- Side-by-side comparisons of experiments.
- Drill-down views to analyze specific runs.
- Visualizations like learning curves, confusion matrices, and ROC curves.
3. Client Library
An interface for logging and querying data programmatically. Libraries like MLflow, Neptune, and Weights & Biases provide APIs for seamless integration with machine learning workflows.
ML Experiment Tracking vs. Model Management
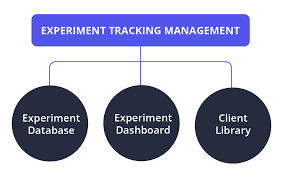
While both are part of the MLOps lifecycle, they serve distinct purposes:
- Experiment Tracking:
- Focuses on the iterative development phase of models.
- Tracks configurations, metrics, and artifacts for all experiments.
- Model Management:
- Begins when a model is ready for production.
- Deals with versioning, deployment, monitoring, and retraining.
Not every experiment leads to production, but robust tracking ensures readiness when models are deployed.
What Should Be Tracked in ML Experiments?
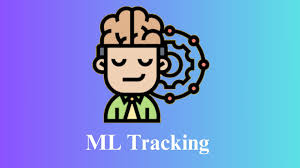
- Code:
- Preprocessing, training, and evaluation scripts.
- Save environment configurations like
requirements.txt
orDockerfile
.
- Data:
- Record dataset versions, file hashes, or paths to ensure data consistency.
- Parameters:
- Capture hyperparameters like learning rate, batch size, and architecture settings.
- Metrics:
- Log evaluation metrics across training, validation, and test sets.
- Artifacts:
- Save model weights, evaluation charts, and prediction examples.
Approaches to Experiment Tracking
1. Manual Tracking with Spreadsheets
- Create a spreadsheet for logging metrics, parameters, and results.
- Use consistent naming conventions for experiment files.
- Challenges:
- Prone to human error.
- Difficult to scale and collaborate on.
2. Versioning with GitHub
- Commit experiment metadata to GitHub repositories.
- Automate logging with post-commit hooks.
- Challenges:
- Git wasn’t designed for comparing ML objects like metrics and learning curves.
3. Modern Experiment Tracking Tools
Tools like MLflow, Weights & Biases, and Neptune are purpose-built for experiment tracking. They provide:
- Centralized databases for metadata.
- Dashboards for visualization and analysis.
- Seamless integration with ML frameworks.
Benefits of Modern Experiment Tracking Tools
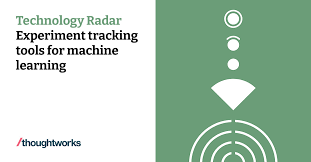
- Centralized Metadata:
- Log experiments in a single repository accessible to the whole team.
- Comparison Features:
- Compare metrics, parameters, and predictions side by side.
- Overlay learning curves and visualize performance differences.
- Advanced Insights:
- Automatically identify impactful parameters.
- Highlight code or data differences across runs.
- Collaboration:
- Share results across teams, enhancing transparency.
Best Practices for Experiment Tracking
- Automate Logging:
- Use tools that automatically capture metadata during training.
- Version Everything:
- Track versions for datasets, models, and configurations.
- Focus on Traceability:
- Ensure every experiment is reproducible.
- Leverage Visual Dashboards:
- Analyze experiments with intuitive visualizations.
Future Trends in Experiment Tracking
- Real-Time Tracking:
- Logging experiment data dynamically during training runs.
- AI-Assisted Tracking:
- Using AI to suggest configurations or highlight anomalies.
- Cloud-Native Solutions:
- Serverless experiment tracking platforms for scalability.
Conclusion
Experiment tracking is a cornerstone of effective machine learning workflows. By centralizing metadata, automating logging, and enabling deep analysis, tracking systems ensure reproducibility, efficiency, and collaboration across projects.
Ready to streamline your ML experiments? Start building your experiment tracking system today!