comprehensive guide to ML Experiment Tracking: Optimizing Machine Learning Workflows 2024
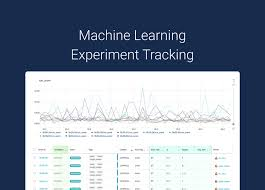
In machine learning, the journey from data to a deployed model involves numerous experiments with varying hyperparameters, datasets, and algorithms. Tracking these experiments effectively is critical for reproducibility, scalability, and collaboration. Without a robust experiment tracking system, valuable insights can be lost, slowing down innovation and increasing time-to-market.
This blog delves deeper into ML experiment tracking, highlighting its significance, components, best practices, and how tools like Neptune.ai revolutionize ML workflows.
What is ML Experiment Tracking?
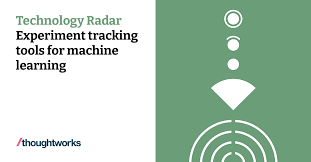
At its core, ML Experiment Tracking involves recording every detail about machine learning experiments to:
- Recreate past results.
- Compare experiments to identify the best-performing models.
- Ensure compliance and maintain audit trails.
Key Features:
- Logging:
- Automatically log hyperparameters, metrics, and results for every experiment.
- Reproducibility:
- Store details like code versions, data references, and training conditions.
- Collaboration:
- Share results and insights across teams in real-time.
Example: Imagine you’re building a sentiment analysis model. By tracking:
- Hyperparameters like learning rates and batch sizes.
- Training metrics like accuracy and loss curves.
- Artifacts like confusion matrices or model weights. You ensure every experiment is reproducible and easy to analyze.
Why is ML Experiment Tracking Important?
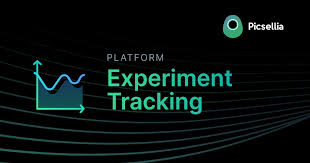
1. Reproducibility
- Problem: Without tracking, it’s nearly impossible to recreate a successful model.
- Solution: Logging configurations, data versions, and results ensures reproducibility.
2. Comparative Analysis
- Problem: Identifying the best model can be challenging without proper comparisons.
- Solution: Tools like Neptune allow you to compare metrics, visualize performance, and find optimal configurations.
3. Scalability
- Problem: Scaling experiments across datasets and hyperparameters leads to metadata overload.
- Solution: Experiment tracking systems organize and store metadata, enabling scalability.
4. Collaboration
- Problem: Teams working in silos struggle to share insights effectively.
- Solution: Centralized dashboards provide a shared platform for review and collaboration.
What to Track in ML Experiments
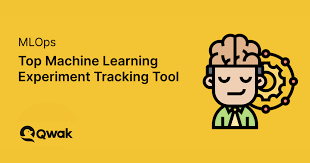
- Code Versions:
- Git commit SHA or snapshot of the training script.
- Data Versions:
- Dataset references, file hashes, or schema versions.
- Hyperparameters:
- Configurations like learning rate, batch size, and optimizer settings.
- Metrics:
- Training and validation metrics like accuracy, F1-score, and loss.
- Artifacts:
- Model checkpoints, visualizations, and prediction examples.
Example in Practice: An e-commerce company building a product recommendation system might track:
- Different recommendation algorithms (e.g., collaborative filtering vs. neural networks).
- Hyperparameter sweeps for embedding size or learning rate.
- Metrics like click-through rate (CTR) and recall.
Best Practices for Experiment Tracking
1. Automate Logging
- Use tools like Neptune.ai or MLflow to automatically capture experiment details.
- Avoid manual logging, which is error-prone and inconsistent.
2. Version Everything
- Track every version of datasets, models, and training code.
- Use tools like DVC for data versioning and Git for code management.
3. Maintain Centralized Repositories
- Store metadata in centralized systems accessible to all team members.
4. Prioritize Collaboration
- Use dashboards to share results and insights.
- Annotate experiments to document findings and rationale.
5. Analyze Trends
- Leverage visualizations to identify trends in metrics across experiments.
Modern Tools for Experiment Tracking
1. Neptune.ai
- Features:
- Logs all metadata automatically.
- Provides cloud-based scalability.
- Offers detailed dashboards for comparison and visualization.
- Use Case: Waabi used Neptune.ai to manage experiments for autonomous vehicle models, scaling seamlessly across thousands of runs.
2. MLflow
- Features:
- Open-source platform for experiment tracking and model management.
- Logs metrics, parameters, and artifacts.
- Use Case: Suitable for teams requiring local deployment with simple workflows.
3. Weights & Biases
- Features:
- Lightweight tracking with strong focus on collaboration.
- Integrated visualizations for learning curves and performance metrics.
- Use Case: Ideal for fast-paced teams needing quick insights.
Challenges in Experiment Tracking
- Metadata Overload:
- Managing large volumes of metadata can overwhelm systems without proper organization.
- Cost Management:
- Storing artifacts like model weights and visualizations can be expensive.
- System Integration:
- Ensuring smooth integration with ML pipelines and tools requires planning.
Emerging Trends in Experiment Tracking
- AI-Driven Insights:
- Systems that recommend optimal hyperparameters or identify anomalies automatically.
- Real-Time Tracking:
- Logging and visualizing metrics during training.
- Cloud-Native Platforms:
- Scalable, serverless solutions that grow with organizational needs.
- End-to-End MLOps Integration:
- Combining tracking with deployment, monitoring, and retraining workflows.
Future of Experiment Tracking
As machine learning continues to evolve, the demand for smarter, more integrated experiment tracking systems will grow. Future solutions will likely incorporate:
- Advanced Visualizations: Interactive 3D visualizations for hyperparameter optimization.
- Seamless Collaboration: AI assistants suggesting insights or improvements in real-time.
- Unified Ecosystems: Platforms that manage the entire ML lifecycle, from experiment tracking to production monitoring.
Conclusion
ML experiment tracking is more than just a tool—it’s a critical enabler for reproducibility, efficiency, and collaboration in machine learning workflows. Whether you’re building models in a research lab or deploying them in production, robust tracking systems like Neptune.ai or MLflow ensure you’re always one step ahead.
Ready to optimize your machine learning experiments? Start tracking smarter today!