comprehensive guide to ML Model Registry: Streamlining Model Lifecycle Management 2024
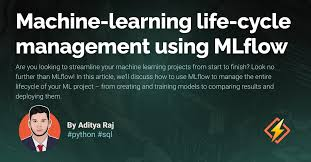
As organizations adopt machine learning at scale, managing models becomes increasingly complex. From experimentation to deployment, teams need robust systems to track, validate, and manage model versions. Enter the ML Model Registry—a centralized repository designed to simplify and organize the entire lifecycle of machine learning models.
This blog explores what an ML Model Registry is, its key features, and how it bridges the gap between experimentation and production.
What is an ML Model Registry?
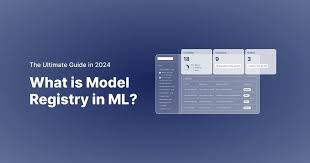
An ML Model Registry is a centralized repository that tracks, manages, and stores machine learning models and their associated metadata. It acts as a single source of truth for all models, enabling seamless collaboration, reproducibility, and efficient deployment.
Key Benefits:
- Centralized Storage:
- Stores models, metadata, and artifacts in one place for easy retrieval.
- Lifecycle Management:
- Tracks models through stages like staging, production, and retirement.
- Collaboration:
- Facilitates better communication between data scientists, engineers, and operations teams.
Model Registry vs. Model Repository
Model Repository
- A storage solution for machine learning models.
- Focuses on saving and retrieving model artifacts.
Model Registry
- A comprehensive system that tracks models, their versions, and lifecycle stages.
- Integrates with pipelines and tools for testing, validation, and deployment.
While both terms are sometimes used interchangeably, a model registry offers advanced features like versioning, metadata tracking, and deployment readiness.
Key Features of an ML Model Registry
1. Centralized Model Storage
- Functionality:
- Stores trained models, weights, configurations, and dependencies.
- Benefits:
- Ensures easy retrieval for auditing, testing, or deployment.
2. Model Versioning
- How It Works:
- Assigns unique versions to models as they are updated.
- Tracks training data versions, code changes, and hyperparameters for each iteration.
- Benefits:
- Enables comparison of model versions to identify the best-performing one.
- Ensures traceability for regulatory compliance.
3. Integration with Experiment Tracking
- Functionality:
- Links experiment management systems with model registration.
- Tracks model training runs, evaluation metrics, and hyperparameters.
- Benefits:
- Provides a complete lineage from experiments to production-ready models.
4. Validation and QA
- Functionality:
- Performs fairness checks, explainability tests, and QA in staging environments.
- Benefits:
- Ensures models meet ethical and business standards before deployment.
5. CI/CD Integration
- Functionality:
- Supports automated pipelines for continuous integration, delivery, and training.
- Uses webhooks or APIs to trigger actions like deployment.
- Benefits:
- Speeds up the model delivery process and reduces manual overhead.
6. Model Deployment and Monitoring
- How It Works:
- Integrates with serving systems to deploy models in production.
- Tracks real-time metrics like latency, throughput, and accuracy.
- Benefits:
- Monitors model performance to ensure reliability and identify drift.
How Does a Model Registry Work?
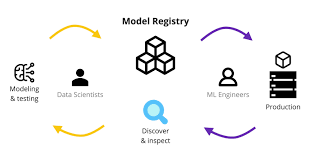
1. Model Registration
- When a model is trained, it is registered in the registry along with metadata:
- Dataset version.
- Training environment and dependencies.
- Validation metrics.
2. Version Control
- Each new version of a model is logged with unique identifiers, enabling teams to:
- Compare model performance.
- Roll back to previous versions if needed.
3. Staging and Deployment
- Models move through various stages:
- Development: Initial experiments and training.
- Staging: Validation and QA testing.
- Production: Deployment for live use cases.
4. Monitoring and Feedback
- Once deployed, models are monitored for performance and drift.
- Metrics are fed back into the registry for continuous improvement.
Challenges Addressed by Model Registries
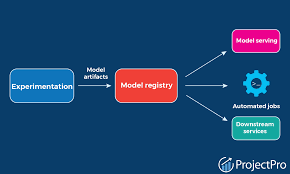
- Model Sprawl:
- Centralizes scattered models across teams and projects.
- Reproducibility:
- Logs training and deployment conditions for each model version.
- Collaboration:
- Provides a unified interface for cross-functional teams.
- Compliance:
- Ensures traceability and auditing for regulated industries.
Popular Tools for Model Registry
1. MLflow Model Registry
- Features:
- Tracks models through staging, production, and retirement.
- Provides APIs for integration with MLOps workflows.
2. Neptune.ai
- Features:
- Offers a centralized dashboard for models and experiments.
- Integrates seamlessly with pipelines.
3. Amazon SageMaker Model Registry
- Features:
- Built for enterprise-scale ML workflows.
- Supports CI/CD integration with SageMaker pipelines.
Best Practices for Using a Model Registry
- Automate Registration:
- Integrate model registration with training pipelines to avoid manual errors.
- Track Metadata:
- Log everything from hyperparameters to model evaluation metrics.
- Leverage Versioning:
- Use version control to ensure reproducibility and easier comparisons.
- Integrate with CI/CD:
- Enable automated pipelines for faster deployment cycles.
- Monitor Deployed Models:
- Continuously track performance metrics to identify drift.
Conclusion
An ML Model Registry is more than just a repository—it’s the backbone of scalable and reproducible machine learning workflows. By providing centralized storage, versioning, and seamless integration with tools, a model registry ensures that models are not only deployed efficiently but also managed effectively throughout their lifecycle.
Ready to streamline your ML model management? Start building smarter workflows with a model registry today!