comprehensive guide to ThunderSVM: A Game-Changer for Accelerating Support Vector Machine Training with GPUs 2024
Support Vector Machines (SVMs) have long been a powerful tool in the machine learning world, excelling in tasks like classification and regression. However, as the amount of data grows, training SVMs can become prohibitively slow. The computational complexity of solving SVM problems increases cubically with respect to the number of training vectors, making it particularly challenging to scale for large datasets.
Enter ThunderSVM, an open-source library designed to speed up the training of SVMs by leveraging GPU and multi-core CPU parallelism. With ThunderSVM, training time can be reduced dramatically, and its optimizations make it suitable for handling big data more efficiently. In this blog, we’ll dive into what ThunderSVM is, how it works, and its practical advantages.
The Challenges of Traditional SVMs
SVMs, despite being powerful, come with several challenges:
- Computational Complexity: The quadratic programming problem at the heart of SVMs scales cubically with the number of training samples, making it computationally expensive.
- Kernel Sensitivity: The choice of kernel significantly affects SVM performance. Finding the best kernel for a specific dataset is not always straightforward.
- Memory-Intensiveness: For large datasets, the kernel matrix can consume substantial memory.
- Slow Training: Training times for SVMs can be long, particularly when dealing with large datasets.
- Limited Scalability: SVMs become impractical for datasets with many features or samples, due to their cubic growth in complexity.
ThunderSVM: Optimizing SVM Performance
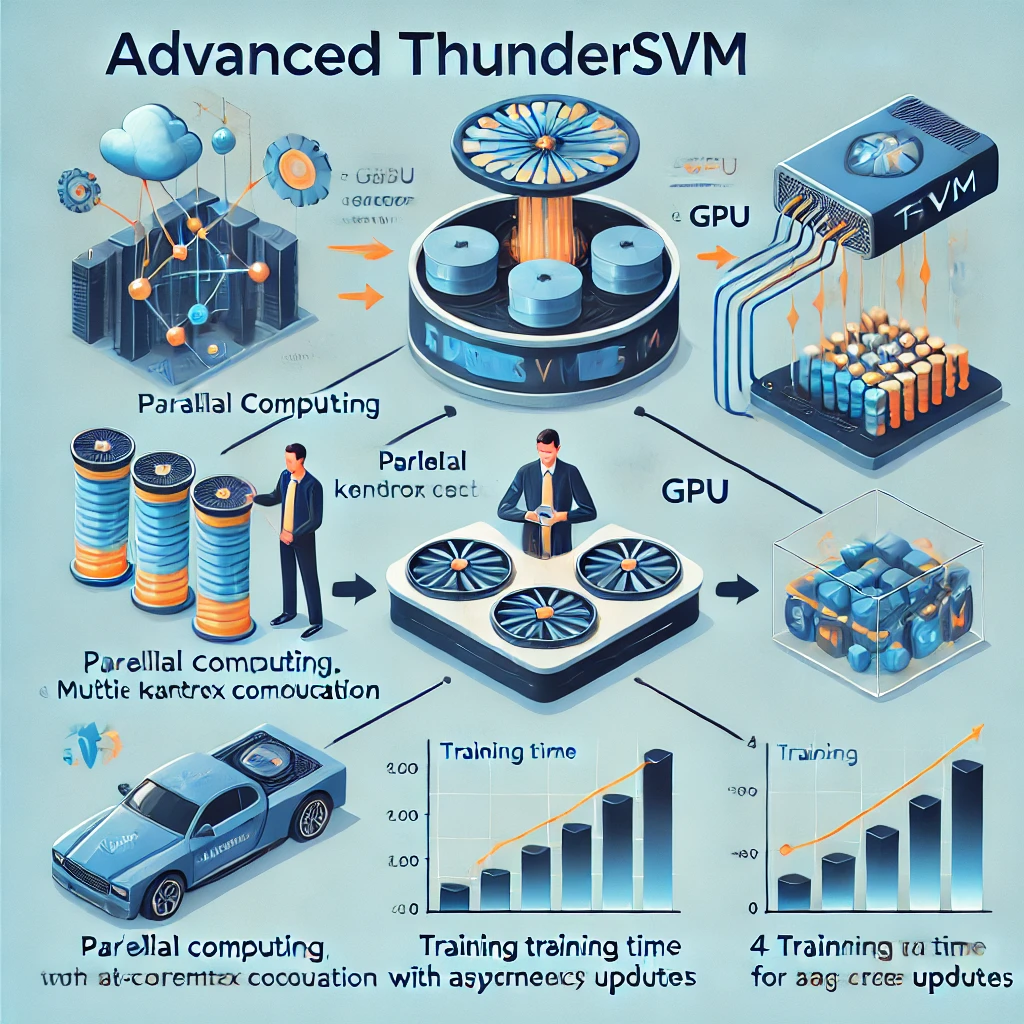
ThunderSVM optimizes SVM training by utilizing parallel computing on GPUs and multi-core CPUs. With ThunderSVM, it’s possible to reduce training time significantly, especially for large datasets. The library provides the following optimizations:
1. Parallelized Sequential Minimal Optimization (SMO)
The SMO algorithm is essential for training SVMs efficiently. ThunderSVM parallelizes SMO in two key steps:
- Step 1 (Parallel Reduction): This step finds two extreme training instances that can improve the SVM the most. ThunderSVM uses parallel reduction to speed this process up, by loading data from GPU memory to shared memory and reducing the array size in parallel.
- Step 2 (Parallel Update): After updating Lagrange multipliers, ThunderSVM parallelizes the update of optimality indicators, dedicating one thread per indicator to maximize parallelism.
2. Efficient Kernel Computation and Optimization
Kernel computations are memory-intensive. ThunderSVM optimizes kernel matrix computation by:
- Batch Kernel Computation: By computing a batch of kernel matrix rows at once and reusing them in GPU memory buffers, ThunderSVM minimizes read/write operations and avoids repeated kernel value computations.
- GPU Shared Memory: ThunderSVM uses GPU shared memory to accelerate parallel reductions and reduce high-latency memory access.
- Matrix Operations: ThunderSVM uses cuSparse, NVIDIA’s high-performance library, for matrix operations to further accelerate kernel computations.
ThunderSVM: Key Advantages
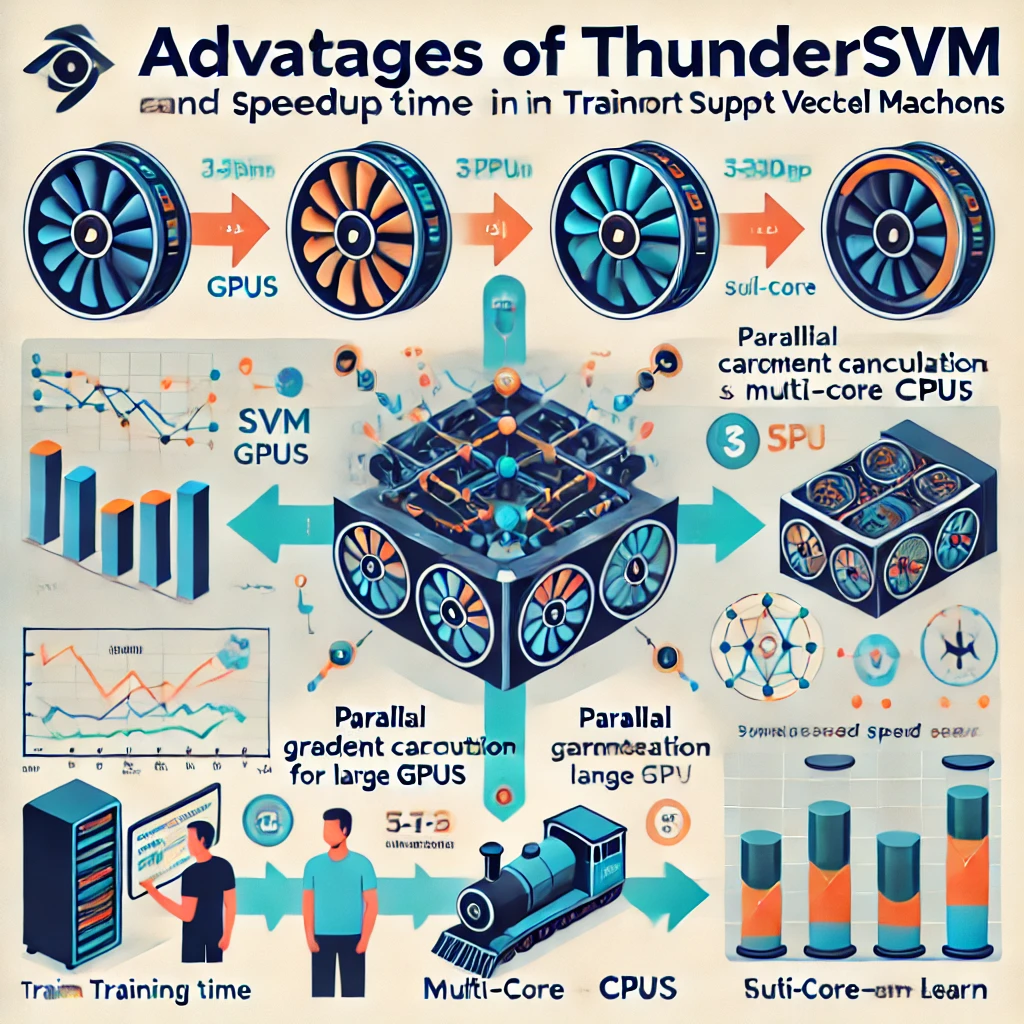
ThunderSVM is designed to provide the following key advantages over traditional SVM implementations:
- Speed: ThunderSVM provides up to 70x faster training compared to scikit-learn’s SVM, especially with large datasets.
- Scalability: ThunderSVM can handle big data efficiently, enabling faster training for large datasets with many features or samples.
- Parallelism: By leveraging both multi-core CPUs and GPUs, ThunderSVM optimizes training performance, reducing computational costs and increasing resource utilization.
- Real-Time Processing: ThunderSVM’s optimizations enable real-time SVM training, a significant benefit for applications that require fast updates to the model.
Practical Implementation with ThunderSVM
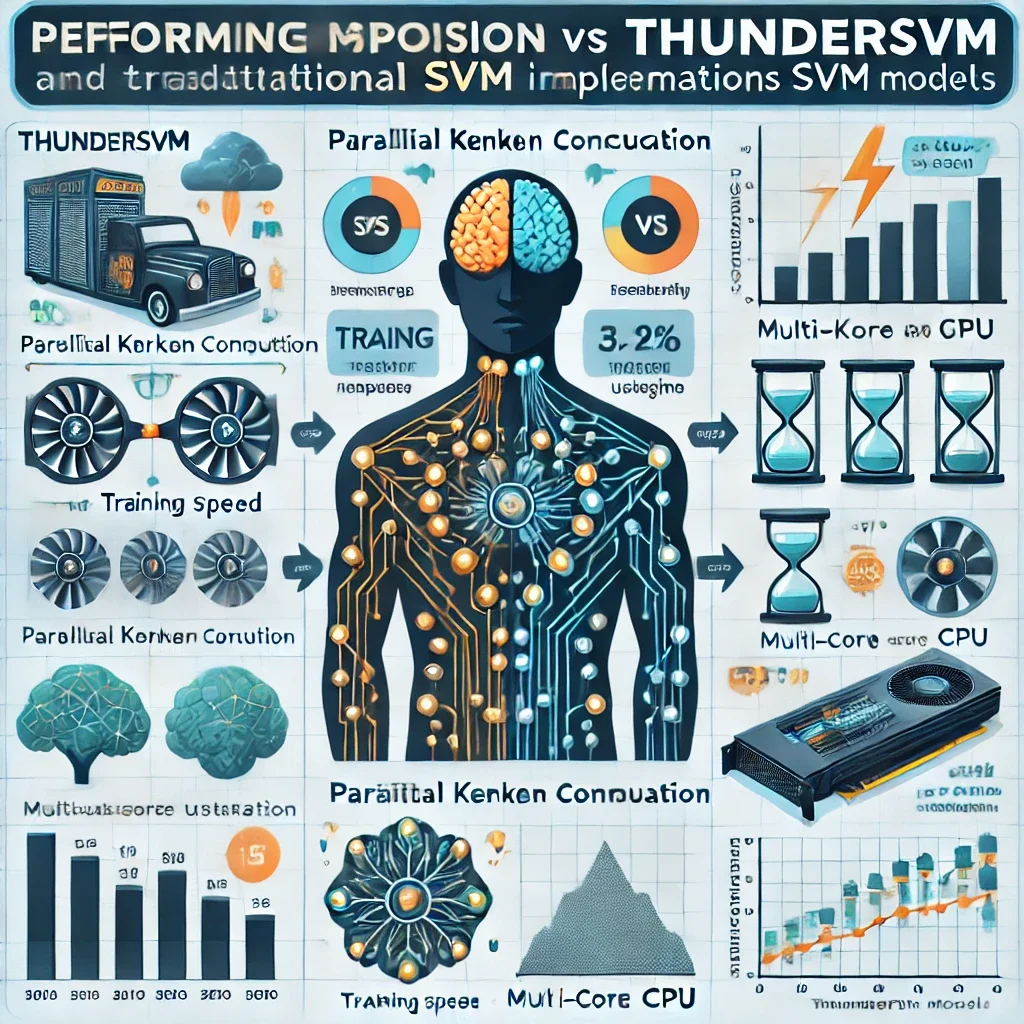
Using ThunderSVM is simple, and you can easily integrate it into your existing code. You can speed up your classification tasks by just changing one line of code.
Here’s a basic example of how to use ThunderSVM for classification:
pythonCopyfrom sklearn.datasets import make_classification
from sklearn.model_selection import train_test_split
from thundersvm import SVC
# Create synthetic dataset
X, y = make_classification(n_samples=100000, n_features=20, random_state=5)
# Split data into training and test sets
X_train, X_test, y_train, y_test = train_test_split(X, y, test_size=0.2, random_state=5)
# Initialize model
model = SVC(C=100, kernel='rbf')
# Train the model
model.fit(X_train, y_train)
# Evaluate accuracy
accuracy = model.score(X_test, y_test)
print(f"Accuracy: {accuracy}")
ThunderSVM Speedup Evaluation
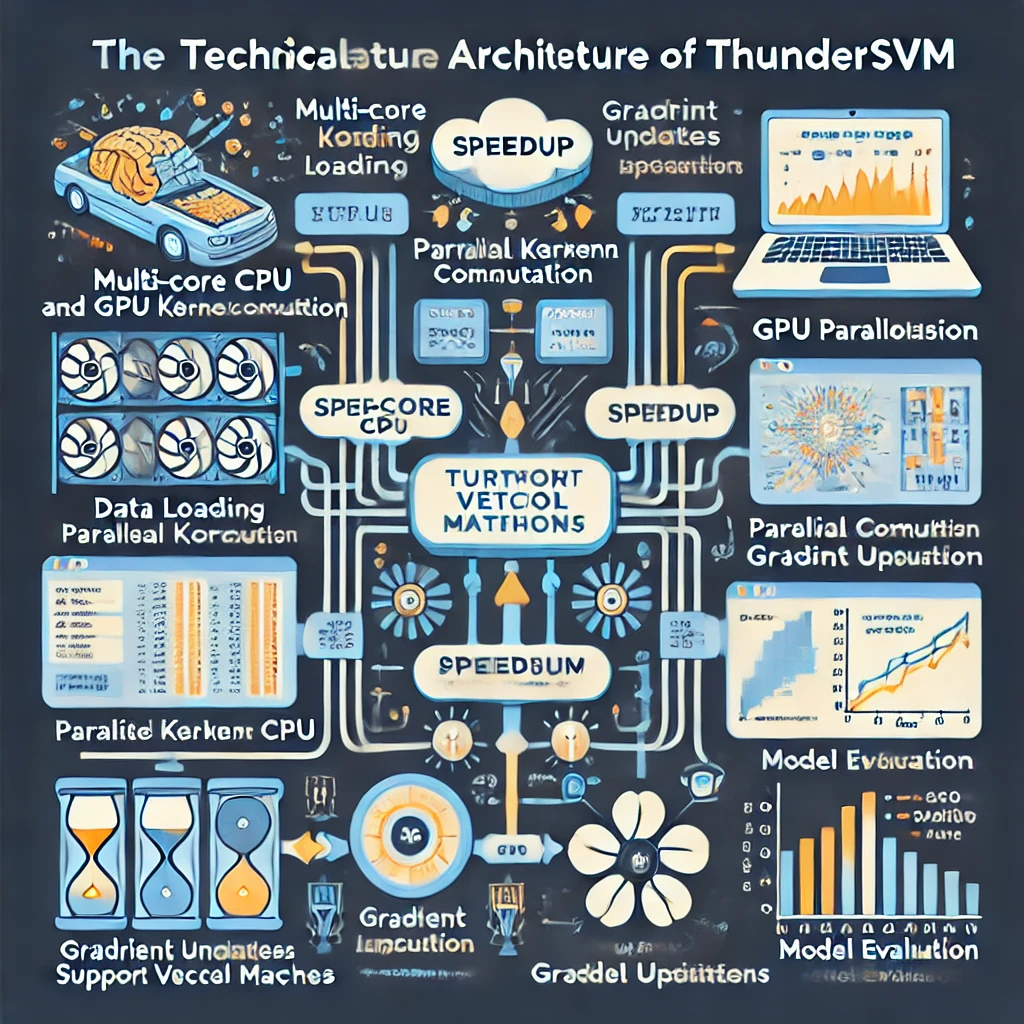
To measure the speedup of ThunderSVM, you can use the following approach, comparing ThunderSVM’s performance against scikit-learn’s SVM:
- Create Synthetic Data: Use
make_classification
to generate a large dataset. - Run scikit-learn’s SVM: Measure the time for training, prediction, and scoring.
- Run ThunderSVM: Similarly, measure the time taken by ThunderSVM for the same task.
Speedup Results Example
pythonCopyfrom sklearn.svm import SVC as skl_SVC
from thundersvm import SVC as ts_SVC
import time
# Timer class to measure elapsed time
class Timer:
def __enter__(self):
self.tick = time.time()
return self
def __exit__(self, *args, **kwargs):
self.tock = time.time()
self.elapsed = self.tock - self.tick
# Initialize datasets
X, y = make_classification(n_samples=100000, n_features=20, random_state=5)
X_train, X_test, y_train, y_test = train_test_split(X, y, test_size=0.2, random_state=5)
# Run scikit-learn SVC
with Timer() as clf_fit:
clf = skl_SVC(C=100)
clf.fit(X_train, y_train)
with Timer() as clf_predict:
clf.predict(X_test)
with Timer() as clf_score:
clf.score(X_test, y_test)
# Print scikit-learn SVC results
print(f"Scikit-learn SVC Time - Fit: {clf_fit.elapsed}s, Predict: {clf_predict.elapsed}s, Score: {clf_score.elapsed}s")
# Run ThunderSVM SVC
with Timer() as model_fit:
model = ts_SVC(C=100)
model.fit(X_train, y_train)
with Timer() as model_predict:
model.predict(X_test)
with Timer() as model_score:
model.score(X_test, y_test)
# Print ThunderSVM results
print(f"ThunderSVM SVC Time - Fit: {model_fit.elapsed}s, Predict: {model_predict.elapsed}s, Score: {model_score.elapsed}s")
ThunderSVM Speedup Example:
textCopyThunderSVM Fit Speedup with 100,000 samples: 56.2x
ThunderSVM Predict Speedup with 100,000 samples: 17.7x
ThunderSVM Score Speedup with 100,000 samples: 17.2x
Conclusion
ThunderSVM is a revolutionary tool for speeding up Support Vector Machine (SVM) training using GPU and multi-core CPUs. With its highly optimized approach, ThunderSVM provides substantial speedups, making it ideal for working with large datasets in real-time applications. Whether you’re working on classification tasks, handling big data, or running models on cloud-based platforms, ThunderSVM can provide the performance boost you need to train your models quickly and efficiently.
By using ThunderSVM, you can unlock the full potential of your hardware and achieve better results with less time and resource consumption, accelerating machine learning workflows and improving overall system performance.