comprehensve guide to Hadoop Architecture: A Foundation for Big Data Processing 2024
In the realm of big data, Apache Hadoop stands as a cornerstone technology that revolutionized how massive datasets are stored and processed. Its distributed architecture ensures scalability, fault tolerance, and efficiency, making it indispensable for organizations dealing with large-scale data.
This blog explores Hadoop’s architecture, its key components, and how it supports big data analytics.
The Conceptual Layers of Hadoop
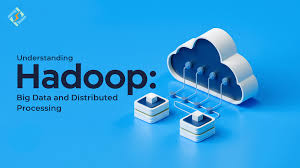
Hadoop’s architecture is designed to handle two critical aspects of big data:
- Data Storage Layer:
- Responsible for storing vast amounts of structured, semi-structured, and unstructured data.
- Built on Hadoop Distributed File System (HDFS).
- Data Processing Layer:
- Handles the computation of stored data using parallel processing techniques.
- Powered by MapReduce or modern frameworks like Apache Spark.
Hadoop High-Level Architecture
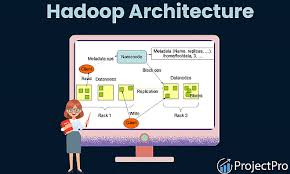
Hadoop follows a Master-Slave Architecture, which consists of the following components:
1. Master Node
The Master Node orchestrates data storage and processing across the Hadoop cluster.
Key Components:
- NameNode (Master HDFS):
- Manages the filesystem namespace.
- Keeps track of file locations and manages metadata for files stored in DataNodes.
- Resource Manager (Master YARN):
- Allocates resources for processing tasks.
- JobTracker (in Hadoop 1.0) or ApplicationMaster (in Hadoop 2.0):
- Assigns and monitors processing tasks across nodes.
2. Slave Node
The Slave Nodes handle data storage and processing tasks assigned by the Master Node.
Key Components:
- DataNode:
- Stores actual data blocks in HDFS.
- Sends periodic heartbeats to the NameNode to confirm availability.
- NodeManager:
- Manages individual tasks and resources on the slave node.
- Executes data processing tasks as instructed by the Resource Manager.
Hadoop Versions: A Comparative Overview
Hadoop 1.0
Features:
- HDFS: A schema-less distributed storage system.
- MapReduce: Processes data using key-value pairs for parallel computation.
Limitations:
- Dependency on MapReduce for all processing.
- Supports only batch processing.
- Inefficient for modern real-time workloads.
Hadoop 2.0
Key Enhancements:
- YARN (Yet Another Resource Navigator):
- A new resource management layer that decouples resource allocation from processing logic.
- Supports any application capable of dividing tasks into parallel processes.
- ApplicationMaster:
- Manages the lifecycle of applications on the Master Node.
- NodeManager:
- Replaces TaskTracker on Slave Nodes for better resource utilization.
Benefits:
- Supports real-time and iterative workloads.
- Removed dependency on MapReduce.
- Improved scalability and flexibility.
Core Components of Hadoop
Hadoop consists of the following four main components:
- Hadoop Common:
- Shared utilities and libraries used by other Hadoop modules.
- HDFS (Hadoop Distributed File System):
- Stores large datasets across multiple nodes with built-in fault tolerance.
- YARN (Yet Another Resource Navigator):
- A resource management layer that allows multiple data processing engines.
- MapReduce:
- A programming model for batch processing of large datasets.
Leading Hadoop Distributions
Several vendors provide enterprise-grade Hadoop distributions with additional tools and support:
- Cloudera: Offers integrated machine learning and analytics tools.
- Hortonworks: Focuses on open-source and community-driven enhancements.
- MapR: Provides high-performance and real-time processing capabilities.
Advantages of Hadoop Architecture
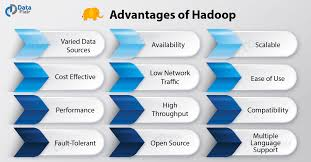
- Scalability:
- Easily scales from a few nodes to thousands.
- Fault Tolerance:
- Data replication ensures availability even if nodes fail.
- Cost-Effectiveness:
- Runs on commodity hardware.
- Flexibility:
- Handles structured, semi-structured, and unstructured data.
- High Throughput:
- Processes large datasets quickly using parallelism.
Real-World Applications of Hadoop
1. Healthcare
- Use Case: Analyzing patient data for personalized treatment plans.
- Impact: Improved patient outcomes and reduced costs.
2. Finance
- Use Case: Fraud detection by analyzing transaction patterns.
- Impact: Faster detection and prevention of fraudulent activities.
3. Retail
- Use Case: Customer segmentation and personalized recommendations.
- Impact: Increased sales and enhanced customer satisfaction.
Challenges of Hadoop Architecture
- Real-Time Processing:
- Hadoop’s batch-oriented approach in Version 1.0 limits real-time analytics.
- Programming Complexity:
- Requires expertise in MapReduce or other compatible frameworks.
- High Latency:
- Heavy reliance on disk I/O can impact performance.
Future of Hadoop Architecture
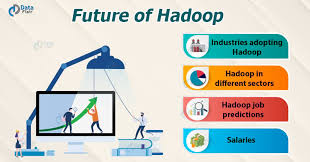
- Integration with Cloud:
- Hadoop is increasingly deployed on cloud platforms like AWS, Azure, and GCP for greater scalability.
- Adoption of Spark:
- Hadoop ecosystems now integrate with Spark for faster, in-memory data processing.
- Edge Analytics:
- Combining Hadoop with edge computing for localized processing and reduced latency.
Conclusion
The Hadoop Architecture is a robust and scalable solution for managing and processing big data. With its distributed storage (HDFS) and parallel processing capabilities (MapReduce), Hadoop has become the backbone of modern data analytics. As organizations continue to deal with growing data complexity, Hadoop’s evolving ecosystem ensures it remains a vital tool in the data-driven landscape.
Are you ready to leverage Hadoop for your big data needs? Start your journey into scalable analytics today!