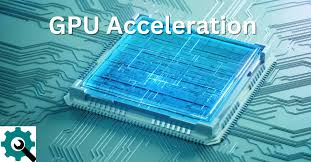
Unlocking the Power of GPUs for Deep Learning: A comprehensive Guide to Accelerating ML Models 2024
In the realm of machine learning (ML), the demand for computational power is growing rapidly, especially with the advent of deep learning (DL). Deep learning models, often comprising billions of parameters, require vast amounts of computational resources to train efficiently. The key to accelerating these models lies in GPU-accelerated computing, which enables parallel processing and significantly speeds up training and inference processes.
In this blog, we will explore the role of GPU acceleration in deep learning, the hardware architecture that powers GPUs, and how to leverage tools like CuPy, Numba, and RAPIDS to maximize the performance of machine learning models.
Why GPUs are Essential for Deep Learning
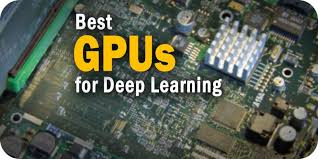
Deep learning models, such as those used in image recognition, natural language processing (NLP), and speech recognition, rely heavily on matrix calculations. These models contain billions of parameters, and training them involves performing billions of matrix operations.
GPUs are well-suited for these tasks because they are designed for parallel processing. Unlike CPUs, which excel at serial tasks, GPUs consist of thousands of smaller cores that can handle many operations simultaneously. This parallelism allows GPUs to process large amounts of data much faster than CPUs, making them ideal for deep learning.
Key GPU Features for Deep Learning
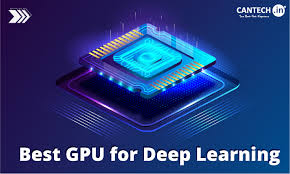
- Tensor Cores: NVIDIA’s tensor cores are specialized processors designed to accelerate deep learning tasks. Tensor cores are optimized for matrix operations, which are central to deep learning. By using tensor cores, NVIDIA GPUs can perform matrix multiplications (common in neural network operations) much faster than traditional CPUs.
- Multi-GPU Clusters: For even larger models, multi-GPU clusters can be employed, where multiple GPUs work together on the same task. Technologies like NVLink and InfiniBand allow GPUs to exchange data directly, bypassing CPU bottlenecks and significantly increasing throughput.
- High Memory Bandwidth: GPUs use specialized memory architectures like HBM (High Bandwidth Memory), which allows them to transfer data between the memory and cores much faster than CPUs. This high memory bandwidth is crucial for training large models where huge datasets need to be processed quickly.
- Efficient Computation with Reduced Precision: GPUs are optimized for mixed-precision training, where lower precision (e.g., FP16) is used to speed up training while maintaining accuracy. This allows GPUs to perform more operations in parallel, enhancing performance.
GPU vs. CPU for Deep Learning
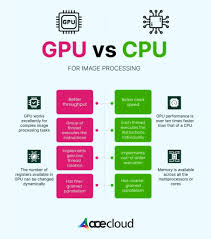
While GPUs are superior in deep learning tasks, CPUs still have their place in certain areas. Here’s a comparison between the two:
- CPUs are better suited for serial task processing, such as handling system operations, running operating systems, and executing tasks that require more complex instruction sets. They typically have fewer cores (2–16) optimized for single-threaded tasks.
- GPUs, on the other hand, excel at parallel processing, with hundreds or even thousands of cores dedicated to simple operations that can be executed simultaneously. This makes them ideal for deep learning, where operations can be parallelized.
Using GPUs for Deep Learning Training
Training deep learning models requires significant computational resources. Here’s how GPU-accelerated training outperforms traditional CPU-based methods:
- Faster Data Transfers: GPUs are designed to handle the large amounts of data required for deep learning. A GPU with 32 GB of HBM can deliver up to 1.2 TBps of memory bandwidth, which is far more than what a CPU can achieve, even with larger amounts of memory.
- Speed: GPUs can speed up training times by a factor of 3x–10x compared to CPUs, depending on the model and dataset size.
- Cost-Effectiveness: Although GPUs are more expensive than CPUs, they are more cost-effective for deep learning tasks because they can drastically reduce training time, which translates to lower costs in cloud environments.
For example, benchmarks show that TensorFlow and Keras models trained on GPU clusters consistently outperform CPU clusters by a significant margin, with throughput improvements ranging from 186% to 804%.
Choosing the Right GPU Configuration
Choosing the optimal GPU configuration for deep learning depends on the stage of the model training and the specific needs of your project. Here are a few factors to consider:
- Memory Size: Deep learning models require large amounts of memory to store parameters and data during training. For instance, training large models like LLaMA with billions of parameters requires GPUs with at least 28 GB of RAM for full precision.
- Tensor Cores: For faster training, choose GPUs with more tensor cores. These cores are designed to handle matrix operations, which are crucial for deep learning.
- Memory Bandwidth: If you are scaling your model across multiple GPUs, memory bandwidth becomes a key factor. Using technologies like NVLink or InfiniBand allows GPUs to communicate more efficiently, which is important for large-scale models.
- Training vs. Inference: The requirements for training and inference differ. Training large models requires high-performance GPUs with plenty of memory and tensor cores. Inference, however, can be performed with less powerful GPUs, depending on the model.
Leveraging RAPIDS and CuPy for GPU-Accelerated Data Science
To fully harness the power of GPUs in data science workflows, NVIDIA offers the RAPIDS suite of libraries. RAPIDS is a collection of open-source software libraries and APIs designed to enable high-performance data science workflows on GPUs. Key components of RAPIDS include:
- CuDF: A GPU-accelerated version of pandas, designed for fast data manipulation.
- CuPy: A GPU-accelerated version of NumPy, optimized for matrix and tensor operations.
- CuML: A GPU-accelerated machine learning library that supports common algorithms like decision trees, k-means clustering, and more.
By integrating RAPIDS with deep learning frameworks like TensorFlow, PyTorch, and MXNet, you can optimize the entire ML pipeline, from data preprocessing to model training and inference.
Framework Interoperability with Zero-Copy Pipelines
For complex workflows, especially when working with multiple libraries, zero-copy pipelines are essential. DLPack and CUDA Array Interface are technologies that enable zero-copy data transfers between different GPU-accelerated frameworks, such as PyTorch, TensorFlow, and RAPIDS. This minimizes the overhead of data conversion and copying, making your pipeline more efficient.
Conclusion: Unlocking the Potential of GPUs for ML
GPU acceleration is a game-changer for deep learning and machine learning. With NVIDIA’s Tensor Cores, multi-GPU clusters, and powerful libraries like RAPIDS, GPUs enable faster training and more efficient data processing, allowing data scientists to work with larger datasets and more complex models. Whether you’re working on image recognition, NLP, or reinforcement learning, GPUs provide the performance boost needed to push the boundaries of what’s possible in ML.
By leveraging tools like CuPy, Numba, and RAPIDS, you can take full advantage of GPU power to accelerate every stage of your machine learning workflow—from data manipulation to model training and inference.